Open Access
ARTICLE
Predicted Oil Recovery Scaling-Law Using Stochastic Gradient Boosting Regression Model
1 Energy Research Lab., College of Engineering, Effat University, Jeddah, 21478, KSA
2 Artificial Intelligence and Cyber Security Research Lab., College of Engineering, Effat University, Jeddah, 21478, KSA
3 Department of Mathematics-College of Sciences & Humanities in Al Aflaj, Prince Sattam bin Abdulaziz University, Al-Aflaj, 11912, KSA
4 Department of Physics, College of Science & Humanities in Al-Aflaj, Prince Sattam bin Abdulaziz University, Al-Aflaj, 11912, KSA
5 Mathematics Department, Faculty of Science, Aswan University, Aswan, 81528, Egypt
* Corresponding Author: Mahmoud M. Selim. Email:
(This article belongs to the Special Issue: Machine Learning-based Intelligent Systems: Theories, Algorithms, and Applications)
Computers, Materials & Continua 2021, 68(2), 2349-2362. https://doi.org/10.32604/cmc.2021.017102
Received 21 January 2021; Accepted 26 February 2021; Issue published 13 April 2021
Abstract
In the process of oil recovery, experiments are usually carried out on core samples to evaluate the recovery of oil, so the numerical data are fitted into a non-dimensional equation called scaling-law. This will be essential for determining the behavior of actual reservoirs. The global non-dimensional time-scale is a parameter for predicting a realistic behavior in the oil field from laboratory data. This non-dimensional universal time parameter depends on a set of primary parameters that inherit the properties of the reservoir fluids and rocks and the injection velocity, which dynamics of the process. One of the practical machine learning (ML) techniques for regression/classification problems is gradient boosting (GB) regression. The GB produces a prediction model as an ensemble of weak prediction models that can be done at each iteration by matching a least-squares base-learner with the current pseudo-residuals. Using a randomization process increases the execution speed and accuracy of GB. Hence in this study, we developed a stochastic regression model of gradient boosting (SGB) to forecast oil recovery. Different non-dimensional time-scales have been used to generate data to be used with machine learning techniques. The SGB method has been found to be the best machine learning technique for predicting the non-dimensional time-scale, which depends on oil/rock properties.Keywords
Cite This Article
Citations
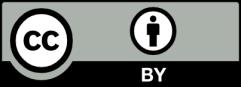
This work is licensed under a Creative Commons Attribution 4.0 International License , which permits unrestricted use, distribution, and reproduction in any medium, provided the original work is properly cited.