Open Access
ARTICLE
Brain Cancer Tumor Classification from Motion-Corrected MRI Images Using Convolutional Neural Network
1 Department of Information Systems, College of Computer and Information Sciences, Princess Nourah bint Abdulrahman University, Riyadh, 11671, Saudi Arabia
2 Department of Computer Science, College of Computer and Information Sciences, Princess Nourah bint Abdulrahman University, Riyadh, 11671, Saudi Arabia
3 Department of Computer and Systems Engineering, Faculty of Engineering, University of Alexandria, Alexandria, Egypt
* Corresponding Author: Hanan Abdullah Mengash. Email:
Computers, Materials & Continua 2021, 68(2), 1551-1563. https://doi.org/10.32604/cmc.2021.016907
Received 15 January 2021; Accepted 19 February 2021; Issue published 13 April 2021
Abstract
Detection of brain tumors in MRI images is the first step in brain cancer diagnosis. The accuracy of the diagnosis depends highly on the expertise of radiologists. Therefore, automated diagnosis of brain cancer from MRI is receiving a large amount of attention. Also, MRI tumor detection is usually followed by a biopsy (an invasive procedure), which is a medical procedure for brain tumor classification. It is of high importance to devise automated methods to aid radiologists in brain cancer tumor diagnosis without resorting to invasive procedures. Convolutional neural network (CNN) is deemed to be one of the best machine learning algorithms to achieve high-accuracy results in tumor identification and classification. In this paper, a CNN-based technique for brain tumor classification has been developed. The proposed CNN can distinguish between normal (no-cancer), astrocytoma tumors, gliomatosis cerebri tumors, and glioblastoma tumors. The implemented CNN was tested on MRI images that underwent a motion-correction procedure. The CNN was evaluated using two performance measurement procedures. The first one is a k-fold cross-validation testing method, in which we tested the dataset using k = 8, 10, 12, and 14. The best accuracy for this procedure was 96.26% when k = 10. To overcome the over-fitting problem that could be occurred in the k-fold testing method, we used a hold-out testing method as a second evaluation procedure. The results of this procedure succeeded in attaining 97.8% accuracy, with a specificity of 99.2% and a sensitivity of 97.32%. With this high accuracy, the developed CNN architecture could be considered an effective automated diagnosis method for the classification of brain tumors from MRI images.Keywords
Cite This Article
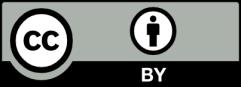