Open Access
ARTICLE
Convolutional Bi-LSTM Based Human Gait Recognition Using Video Sequences
1 University of Wah, Wah Cantt, 47040, Pakistan
2 National University of Technology (NUTECH), Islamabad, 44000, Pakistan
3 COMSATS University Islamabad, Wah Campus, Wah Cantt, Pakistan
4 Faculty of Applied Computing and Technology, Noroff University College, Kristiansand, Norway
5 Department of Computer Science and Engineering, Soonchunhyang University, Asan, 31538, Korea
6 Department of Mathematics, University of Leicester, Leicester, UK
* Corresponding Author: Yunyoung Nam. Email:
Computers, Materials & Continua 2021, 68(2), 2693-2709. https://doi.org/10.32604/cmc.2021.016871
Received 12 January 2021; Accepted 14 February 2021; Issue published 13 April 2021
Abstract
Recognition of human gait is a difficult assignment, particularly for unobtrusive surveillance in a video and human identification from a large distance. Therefore, a method is proposed for the classification and recognition of different types of human gait. The proposed approach is consisting of two phases. In phase I, the new model is proposed named convolutional bidirectional long short-term memory (Conv-BiLSTM) to classify the video frames of human gait. In this model, features are derived through convolutional neural network (CNN) named ResNet-18 and supplied as an input to the LSTM model that provided more distinguishable temporal information. In phase II, the YOLOv2-squeezeNet model is designed, where deep features are extricated using the fireconcat-02 layer and fed/passed to the tinyYOLOv2 model for recognized/localized the human gaits with predicted scores. The proposed method achieved up to 90% correct prediction scores on CASIA-A, CASIA-B, and the CASIA-C benchmark datasets. The proposed method achieved better/improved prediction scores as compared to the recent existing works.Keywords
Cite This Article
Citations
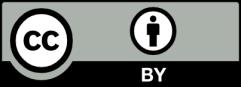
This work is licensed under a Creative Commons Attribution 4.0 International License , which permits unrestricted use, distribution, and reproduction in any medium, provided the original work is properly cited.