Open Access
ARTICLE
Emotion Analysis: Bimodal Fusion of Facial Expressions and EEG
1 Brain Cognitive Computing Lab, School of Information Engineering, Minzu University of China, Beijing, 100081, China
2 Case Western Reserve University, USA
* Corresponding Author: Huiping Jiang. Email:
Computers, Materials & Continua 2021, 68(2), 2315-2327. https://doi.org/10.32604/cmc.2021.016832
Received 13 January 2021; Accepted 24 February 2021; Issue published 13 April 2021
Abstract
With the rapid development of deep learning and artificial intelligence, affective computing, as a branch field, has attracted increasing research attention. Human emotions are diverse and are directly expressed via non-physiological indicators, such as electroencephalogram (EEG) signals. However, whether emotion-based or EEG-based, these remain single-modes of emotion recognition. Multi-mode fusion emotion recognition can improve accuracy by utilizing feature diversity and correlation. Therefore, three different models have been established: the single-mode-based EEG-long and short-term memory (LSTM) model, the Facial-LSTM model based on facial expressions processing EEG data, and the multi-mode LSTM-convolutional neural network (CNN) model that combines expressions and EEG. Their average classification accuracy was 86.48%, 89.42%, and 93.13%, respectively. Compared with the EEG-LSTM model, the Facial-LSTM model improved by about 3%. This indicated that the expression mode helped eliminate EEG signals that contained few or no emotional features, enhancing emotion recognition accuracy. Compared with the Facial-LSTM model, the classification accuracy of the LSTM-CNN model improved by 3.7%, showing that the addition of facial expressions affected the EEG features to a certain extent. Therefore, using various modal features for emotion recognition conforms to human emotional expression. Furthermore, it improves feature diversity to facilitate further emotion recognition research.Keywords
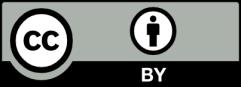