Open Access
ARTICLE
An Optimal Classification Model for Rice Plant Disease Detection
1 Department of Electronics and Communication Engineering, University College of Engineering, BIT Campus, Anna University, Tiruchirappalli, 620024, India
2 Department of Electronics and Communication Engineering, Gokaraju Rangaraju Institute of Engineering and Technology, Hyderabad, 500090, India
3 Department of Electronics and Communication Engineering, Karpagam Academy of Higher Education, Coimbatore, 641021, India
4 Department of Entrepreneurship and Logistics, Plekhanov Russian University of Economics, Moscow, 117997, Russia
5 Department of Logistics, State University of Management, Moscow, 109542, Russia
6 Department of Computer Applications, Alagappa University, Karaikudi, 630001, India
* Corresponding Author: T. Jayasankar. Email:
Computers, Materials & Continua 2021, 68(2), 1751-1767. https://doi.org/10.32604/cmc.2021.016825
Received 13 January 2021; Accepted 17 February 2021; Issue published 13 April 2021
Abstract
Internet of Things (IoT) paves a new direction in the domain of smart farming and precision agriculture. Smart farming is an upgraded version of agriculture which is aimed at improving the cultivation practices and yield to a certain extent. In smart farming, IoT devices are linked among one another with new technologies to improve the agricultural practices. Smart farming makes use of IoT devices and contributes in effective decision making. Rice is the major food source in most of the countries. So, it becomes inevitable to detect rice plant diseases during early stages with the help of automated tools and IoT devices. The development and application of Deep Learning (DL) models in agriculture offers a way for early detection of rice diseases and increase the yield and profit. This study presents a new Convolutional Neural Network-based inception with ResNset v2 model and Optimal Weighted Extreme Learning Machine (CNNIR-OWELM)-based rice plant disease diagnosis and classification model in smart farming environment. The proposed CNNIR-OWELM method involves a set of IoT devices which capture the images of rice plants and transmit it to cloud server via internet. The CNNIR-OWELM method uses histogram segmentation technique to determine the affected regions in rice plant image. In addition, a DL-based inception with ResNet v2 model is engaged to extract the features. Besides, in OWELM, the Weighted Extreme Learning Machine (WELM), optimized by Flower Pollination Algorithm (FPA), is employed for classification purpose. The FPA is incorporated into WELM to determine the optimal parameters such as regularization coefficient C and kernel . The outcome of the presented model was validated against a benchmark image dataset and the results were compared with one another. The simulation results inferred that the presented model effectively diagnosed the disease with high sensitivity of 0.905, specificity of 0.961, and accuracy of 0.942.Keywords
Cite This Article
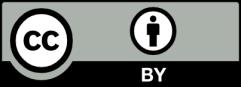
This work is licensed under a Creative Commons Attribution 4.0 International License , which permits unrestricted use, distribution, and reproduction in any medium, provided the original work is properly cited.