Open Access
ARTICLE
Accurate and Computational Efficient Joint Multiple Kronecker Pursuit for Tensor Data Recovery
1 Guangdong Key Laboratory of Intelligent Information Processing, College of Electronics and Information Engineering, Shenzhen University, Shenzhen, 518061, China
2 Department of Housing and Public Works, Queensland, Australia
3 Customer Service Centre, Guangdong Power Grid Corporation, Guangzhou, China
* Corresponding Author: Peng Zhang. Email:
Computers, Materials & Continua 2021, 68(2), 2111-2126. https://doi.org/10.32604/cmc.2021.016804
Received 12 January 2021; Accepted 17 February 2021; Issue published 13 April 2021
Abstract
This paper addresses the problem of tensor completion from limited samplings. Generally speaking, in order to achieve good recovery result, many tensor completion methods employ alternative optimization or minimization with SVD operations, leading to a high computational complexity. In this paper, we aim to propose algorithms with high recovery accuracy and moderate computational complexity. It is shown that the data to be recovered contains structure of Kronecker Tensor decomposition under multiple patterns, and therefore the tensor completion problem becomes a Kronecker rank optimization one, which can be further relaxed into tensor Frobenius-norm minimization with a constraint of a maximum number of rank-1 basis or tensors. Then the idea of orthogonal matching pursuit is employed to avoid the burdensome SVD operations. Based on these, two methods, namely iterative rank-1 tensor pursuit and joint rank-1 tensor pursuit are proposed. Their economic variants are also included to further reduce the computational and storage complexity, making them effective for large-scale data tensor recovery. To verify the proposed algorithms, both synthesis data and real world data, including SAR data and video data completion, are used. Comparing to the single pattern case, when multiple patterns are used, more stable performance can be achieved with higher complexity by the proposed methods. Furthermore, both results from synthesis and real world data shows the advantage of the proposed methods in term of recovery accuracy and/or computational complexity over the state-of-the-art methods. To conclude, the proposed tensor completion methods are suitable for large scale data completion with high recovery accuracy and moderate computational complexity.Keywords
Cite This Article
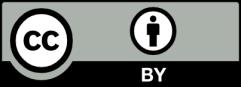