Open Access
ARTICLE
VGG-CovidNet: Bi-Branched Dilated Convolutional Neural Network for Chest X-Ray-Based COVID-19 Predictions
1 Information Systems, King Abdulaziz University, Jeddah, Saudi Arabia
2 Department of Science, Umm Al Qura University, Mecca, Saudi Arabia
* Corresponding Author: Muhammed Binsawad. Email:
(This article belongs to the Special Issue: AI, IoT, Blockchain Assisted Intelligent Solutions to Medical and Healthcare Systems)
Computers, Materials & Continua 2021, 68(2), 2791-2806. https://doi.org/10.32604/cmc.2021.016141
Received 24 December 2020; Accepted 27 February 2021; Issue published 13 April 2021
Abstract
The coronavirus disease 2019 (COVID-19) pandemic has had a devastating impact on the health and welfare of the global population. A key measure to combat COVID-19 has been the effective screening of infected patients. A vital screening process is the chest radiograph. Initial studies have shown irregularities in the chest radiographs of COVID-19 patients. The use of the chest X-ray (CXR), a leading diagnostic technique, has been encouraged and driven by several ongoing projects to combat this disease because of its historical effectiveness in providing clinical insights on lung diseases. This study introduces a dilated bi-branched convoluted neural network (CNN) architecture, VGG-COVIDNet, to detect COVID-19 cases from CXR images. The front end of the VGG-COVIDNet consists of the first 10 layers of VGG-16, where the convolutional layers in these layers are reduced to two to minimize latency during the training phase. The last two branches of the proposed architecture consist of dilated convolutional layers to reduce the model’s computational complexity while retaining the feature maps’ spatial information. The simulation results show that the proposed architecture is superior to all the state-of-the-art architecture in accuracy and sensitivity. The proposed architecture’s accuracy and sensitivity are 96.5% and 96%, respectively, for each infection type.Keywords
Cite This Article
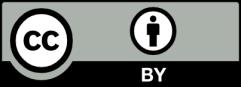