Open Access
ARTICLE
Ozone Depletion Identification in Stratosphere Through Faster Region-Based Convolutional Neural Network
1 Department of Computer Sciences, Kinnaird College for Women, Lahore, 54000, Pakistan
2 Physics Department, College of Science, Jouf University, Sakaka, Aljouf, 72341, Saudi Arabia
3 Department of Basic Sciences, Deanship of Common First Year, Jouf University, Sakaka, Aljouf, 72341, Saudi Arabia
* Corresponding Author: Fahad Ahmad. Email:
Computers, Materials & Continua 2021, 68(2), 2159-2178. https://doi.org/10.32604/cmc.2021.015922
Received 14 December 2020; Accepted 26 February 2021; Issue published 13 April 2021
Abstract
The concept of classification through deep learning is to build a model that skillfully separates closely-related images dataset into different classes because of diminutive but continuous variations that took place in physical systems over time and effect substantially. This study has made ozone depletion identification through classification using Faster Region-Based Convolutional Neural Network (F-RCNN). The main advantage of F-RCNN is to accumulate the bounding boxes on images to differentiate the depleted and non-depleted regions. Furthermore, image classification’s primary goal is to accurately predict each minutely varied case’s targeted classes in the dataset based on ozone saturation. The permanent changes in climate are of serious concern. The leading causes beyond these destructive variations are ozone layer depletion, greenhouse gas release, deforestation, pollution, water resources contamination, and UV radiation. This research focuses on the prediction by identifying the ozone layer depletion because it causes many health issues, e.g., skin cancer, damage to marine life, crops damage, and impacts on living being’s immune systems. We have tried to classify the ozone images dataset into two major classes, depleted and non-depleted regions, to extract the required persuading features through F-RCNN. Furthermore, CNN has been used for feature extraction in the existing literature, and those extricated diverse RoIs are passed on to the CNN for grouping purposes. It is difficult to manage and differentiate those RoIs after grouping that negatively affects the gathered results. The classification outcomes through F-RCNN approach are proficient and demonstrate that general accuracy lies between 91% to 93% in identifying climate variation through ozone concentration classification, whether the region in the image under consideration is depleted or non-depleted. Our proposed model presented 93% accuracy, and it outperforms the prevailing techniques.Keywords
Cite This Article
Citations
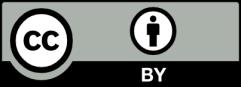
This work is licensed under a Creative Commons Attribution 4.0 International License , which permits unrestricted use, distribution, and reproduction in any medium, provided the original work is properly cited.