Open Access
ARTICLE
Gastric Tract Disease Recognition Using Optimized Deep Learning Features
1 Department of Computer Science, HITEC University, Taxila, 47040, Pakistan
2 Department of Computer Engineering, College of Computer and Information Sciences, King Saud University, Riyadh, 11543, Saudi Arabia
3 Department of Computer Science and Engineering, Soonchunhyang University, Asan, Korea
4 Department of Mathematics and Computer Science, Faculty of Science, Beirut Arab University, Beirut, Lebanon
* Corresponding Author: Yunyoung Nam. Email:
(This article belongs to the Special Issue: Artificial Intelligence and IoT based intelligent systems using high performance computing for Medical applications.)
Computers, Materials & Continua 2021, 68(2), 2041-2056. https://doi.org/10.32604/cmc.2021.015916
Received 14 December 2020; Accepted 13 February 2021; Issue published 13 April 2021
Abstract
Artificial intelligence aids for healthcare have received a great deal of attention. Approximately one million patients with gastrointestinal diseases have been diagnosed via wireless capsule endoscopy (WCE). Early diagnosis facilitates appropriate treatment and saves lives. Deep learning-based techniques have been used to identify gastrointestinal ulcers, bleeding sites, and polyps. However, small lesions may be misclassified. We developed a deep learning-based best-feature method to classify various stomach diseases evident in WCE images. Initially, we use hybrid contrast enhancement to distinguish diseased from normal regions. Then, a pretrained model is fine-tuned, and further training is done via transfer learning. Deep features are extracted from the last two layers and fused using a vector length-based approach. We improve the genetic algorithm using a fitness function and kurtosis to select optimal features that are graded by a classifier. We evaluate a database containing 24,000 WCE images of ulcers, bleeding sites, polyps, and healthy tissue. The cubic support vector machine classifier was optimal; the average accuracy was 99%.Keywords
Cite This Article
Citations
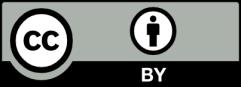
This work is licensed under a Creative Commons Attribution 4.0 International License , which permits unrestricted use, distribution, and reproduction in any medium, provided the original work is properly cited.