Open Access
ARTICLE
Black Hole and Sink Hole Attack Detection in Wireless Body Area Networks
1 School of Computing Science and Engineering, Galgotias University, 201310, India
2 Department of Information Technology, Kongu Engineering College, Erode, 638060, India
3 Department of Health and Human Development, Stefan cel Mare University, Romania
4 Department of Computers, Electronics and Automation, Stefan cel Mare University, Romania
* Corresponding Author: Oana Geman. Email:
Computers, Materials & Continua 2021, 68(2), 1949-1965. https://doi.org/10.32604/cmc.2021.015363
Received 17 November 2020; Accepted 08 February 2021; Issue published 13 April 2021
Abstract
In Wireless Body Area Networks (WBANs) with respect to health care, sensors are positioned inside the body of an individual to transfer sensed data to a central station periodically. The great challenges posed to healthcare WBANs are the black hole and sink hole attacks. Data from deployed sensor nodes are attracted by sink hole or black hole nodes while grabbing the shortest path. Identifying this issue is quite a challenging task as a small variation in medicine intake may result in a severe illness. This work proposes a hybrid detection framework for attacks by applying a Proportional Coinciding Score (PCS) and an MK-Means algorithm, which is a well-known machine learning technique used to raise attack detection accuracy and decrease computational difficulties while giving treatments for heartache and respiratory issues. First, the gathered training data feature count is reduced through data pre-processing in the PCS. Second, the pre-processed features are sent to the MK-Means algorithm for training the data and promoting classification. Third, certain attack detection measures given by the intrusion detection system, such as the number of data packages trans-received, are identified by the MK-Means algorithm. This study demonstrates that the MK-Means framework yields a high detection accuracy with a low packet loss rate, low communication overhead, and reduced end-to-end delay in the network and improves the accuracy of biomedical data.Keywords
Cite This Article
Citations
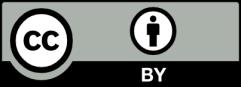
This work is licensed under a Creative Commons Attribution 4.0 International License , which permits unrestricted use, distribution, and reproduction in any medium, provided the original work is properly cited.