Open Access
ARTICLE
Machine Learning Techniques Applied to Electronic Healthcare Records to Predict Cancer Patient Survivability
1 eVIDA Lab, University of Deusto, Bilbao, 48007, Spain
2 Success Clinic Oy, Helsinki, 00180, Finland
* Corresponding Author: Ornela Bardhi. Email:
(This article belongs to the Special Issue: AI, IoT, Blockchain Assisted Intelligent Solutions to Medical and Healthcare Systems)
Computers, Materials & Continua 2021, 68(2), 1595-1613. https://doi.org/10.32604/cmc.2021.015326
Received 15 November 2020; Accepted 06 February 2021; Issue published 13 April 2021
Abstract
Breast cancer (BCa) and prostate cancer (PCa) are the two most common types of cancer. Various factors play a role in these cancers, and discovering the most important ones might help patients live longer, better lives. This study aims to determine the variables that most affect patient survivability, and how the use of different machine learning algorithms can assist in such predictions. The AURIA database was used, which contains electronic healthcare records (EHRs) of 20,006 individual patients diagnosed with either breast or prostate cancer in a particular region in Finland. In total, there were 178 features for BCa and 143 for PCa. Six feature selection algorithms were used to obtain the 21 most important variables for BCa, and 19 for PCa. These features were then used to predict patient survivability by employing nine different machine learning algorithms. Seventy-five percent of the dataset was used to train the models and 25% for testing. Cross-validation was carried out using the StratifiedKfold technique to test the effectiveness of the machine learning models. The support vector machine classifier yielded the best ROC with an area under the curve (AUC) = 0.83, followed by the KNeighborsClassifier with AUC = 0.82 for the BCa dataset. The two algorithms that yielded the best results for PCa are the random forest classifier and KNeighborsClassifier, both with AUC = 0.82. This study shows that not all variables are decisive when predicting breast or prostate cancer patient survivability. By narrowing down the input variables, healthcare professionals were able to focus on the issues that most impact patients, and hence devise better, more individualized care plans.Keywords
Cite This Article
Citations
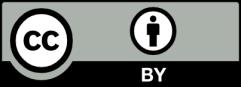
This work is licensed under a Creative Commons Attribution 4.0 International License , which permits unrestricted use, distribution, and reproduction in any medium, provided the original work is properly cited.