Open Access
ARTICLE
Uncertainty Analysis on Electric Power Consumption
1 University College, Sungkyunkwan University, Seoul, 03063, Korea
2 Department of Computer Education, Sungkyunkwan University, Seoul, 03063, Korea
* Corresponding Author: Jaehyoun Kim. Email:
Computers, Materials & Continua 2021, 68(2), 2621-2632. https://doi.org/10.32604/cmc.2021.014665
Received 07 October 2020; Accepted 15 February 2021; Issue published 13 April 2021
Abstract
The analysis of large time-series datasets has profoundly enhanced our ability to make accurate predictions in many fields. However, unpredictable phenomena, such as extreme weather events or the novel coronavirus 2019 (COVID-19) outbreak, can greatly limit the ability of time-series analyses to establish reliable patterns. The present work addresses this issue by applying uncertainty analysis using a probability distribution function, and applies the proposed scheme within a preliminary study involving the prediction of power consumption for a single hotel in Seoul, South Korea based on an analysis of 53,567 data items collected by the Korea Electric Power Corporation using robotic process automation. We first apply Facebook Prophet for conducting time-series analysis. The results demonstrate that the COVID-19 outbreak seriously compromised the reliability of the time-series analysis. Then, machine learning models are developed in the TensorFlow framework for conducting uncertainty analysis based on modeled relationships between electric power consumption and outdoor temperature. The benefits of the proposed uncertainty analysis for predicting the electricity consumption of the hotel building are demonstrated by comparing the results obtained when considering no uncertainty, aleatory uncertainty, epistemic uncertainty, and mixed aleatory and epistemic uncertainty. The minimum and maximum ranges of predicted electricity consumption are obtained when using mixed uncertainty. Accordingly, the application of uncertainty analysis using a probability distribution function greatly improved the predictive power of the analysis compared to time-series analysis.Keywords
Cite This Article
Citations
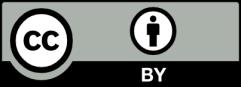
This work is licensed under a Creative Commons Attribution 4.0 International License , which permits unrestricted use, distribution, and reproduction in any medium, provided the original work is properly cited.