Open Access
ARTICLE
Spatial-Resolution Independent Object Detection Framework for Aerial Imagery
1 Deptartment of CSA, Utkal University, Bhubaneswar, 751004, India
2 Department of Information Technology, VNRVJIET, Hyderabad, 500090, India
3 Department of CSE, Sona College of Technology, Salem, 636005, India
4 Research Institute for Innovation & Technology in Education (UNIR iTED), Universidad Internacional de La Rioja (UNIR), Logroño, 26006, Spain
* Corresponding Author: Daniel Burgos. Email:
(This article belongs to the Special Issue: Deep Learning Trends in Intelligent Systems)
Computers, Materials & Continua 2021, 68(2), 1937-1948. https://doi.org/10.32604/cmc.2021.014406
Received 18 September 2020; Accepted 14 February 2021; Issue published 13 April 2021
Abstract
Earth surveillance through aerial images allows more accurate identification and characterization of objects present on the surface from space and airborne platforms. The progression of deep learning and computer vision methods and the availability of heterogeneous multispectral remote sensing data make the field more fertile for research. With the evolution of optical sensors, aerial images are becoming more precise and larger, which leads to a new kind of problem for object detection algorithms. This paper proposes the “Sliding Region-based Convolutional Neural Network (SRCNN),” which is an extension of the Faster Region-based Convolutional Neural Network (RCNN) object detection framework to make it independent of the image’s spatial resolution and size. The sliding box strategy is used in the proposed model to segment the image while detecting. The proposed framework outperforms the state-of-the-art Faster RCNN model while processing images with significantly different spatial resolution values. The SRCNN is also capable of detecting objects in images of any size.Keywords
Cite This Article
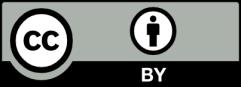
This work is licensed under a Creative Commons Attribution 4.0 International License , which permits unrestricted use, distribution, and reproduction in any medium, provided the original work is properly cited.