Open Access
ARTICLE
Diagnosis of COVID-19 Infection Using Three-Dimensional Semantic Segmentation and Classification of Computed Tomography Images
1 Department of Computer Science, University of Wah, 47040, Pakistan
2 Department of Computer Science, Comsats University Islamabad, Wah Campus, 47040, Pakistan
3 College of Electrical and Mechanical Engineering, National University of Sciences & Technology (NUST), Islamabad, 44000, Pakistan
4 Department of Computer Science and Engineering, Soonchunhyang University, Asan, 31538, Korea
5 Department of Mathematics and Computer Science, Faculty of Science, Beirut Arab University, 115020, Lebanon
6 Faculty of Information Technology, Monash University, Clayton, Victoria, 3800, Australia
* Corresponding Author: Yunyoung Nam. Email:
(This article belongs to the Special Issue: AI, IoT, Blockchain Assisted Intelligent Solutions to Medical and Healthcare Systems)
Computers, Materials & Continua 2021, 68(2), 2451-2467. https://doi.org/10.32604/cmc.2021.014199
Received 16 September 2020; Accepted 10 February 2021; Issue published 13 April 2021
Abstract
Coronavirus 19 (COVID-19) can cause severe pneumonia that may be fatal. Correct diagnosis is essential. Computed tomography (CT) usefully detects symptoms of COVID-19 infection. In this retrospective study, we present an improved framework for detection of COVID-19 infection on CT images; the steps include pre-processing, segmentation, feature extraction/fusion/selection, and classification. In the pre-processing phase, a Gabor wavelet filter is applied to enhance image intensities. A marker-based, watershed controlled approach with thresholding is used to isolate the lung region. In the segmentation phase, COVID-19 lesions are segmented using an encoder-/decoder-based deep learning model in which deepLabv3 serves as the bottleneck and mobilenetv2 as the classification head. DeepLabv3 is an effective decoder that helps to refine segmentation of lesion boundaries. The model was trained using fine-tuned hyperparameters selected after extensive experimentation. Subsequently, the Gray Level Co-occurrence Matrix (GLCM) features and statistical features including circularity, area, and perimeters were computed for each segmented image. The computed features were serially fused and the best features (those that were optimally discriminatory) selected using a Genetic Algorithm (GA) for classification. The performance of the method was evaluated using two benchmark datasets: The COVID-19 Segmentation and the POF Hospital datasets. The results were better than those of existing methods.Keywords
Cite This Article
Citations
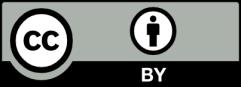
This work is licensed under a Creative Commons Attribution 4.0 International License , which permits unrestricted use, distribution, and reproduction in any medium, provided the original work is properly cited.