Open Access
ARTICLE
Learning Unitary Transformation by Quantum Machine Learning Model
1 School of Information and Software Engineering, University of Electronic Science and Technology of China, Chengdu, 610054, China
2 School of Physics, University of Electronic Science and Technology of China, Chengdu, 610054, China
3 Department of Chemistry, Physics and Atmospheric Sciences, Jackson State University, Jackson, MS, 39217, USA
* Corresponding Author: Xiao-Yu Li. Email:
Computers, Materials & Continua 2021, 68(1), 789-803. https://doi.org/10.32604/cmc.2021.016663
Received 08 January 2021; Accepted 09 February 2021; Issue published 22 March 2021
Abstract
Quantum machine learning (QML) is a rapidly rising research field that incorporates ideas from quantum computing and machine learning to develop emerging tools for scientific research and improving data processing. How to efficiently control or manipulate the quantum system is a fundamental and vexing problem in quantum computing. It can be described as learning or approximating a unitary operator. Since the success of the hybrid-based quantum machine learning model proposed in recent years, we investigate to apply the techniques from QML to tackle this problem. Based on the Choi–Jamiołkowski isomorphism in quantum computing, we transfer the original problem of learning a unitary operator to a min–max optimization problem which can also be viewed as a quantum generative adversarial network. Besides, we select the spectral norm between the target and generated unitary operators as the regularization term in the loss function. Inspired by the hybrid quantum-classical framework widely used in quantum machine learning, we employ the variational quantum circuit and gradient descent based optimizers to solve the min-max optimization problem. In our numerical experiments, the results imply that our proposed method can successfully approximate the desired unitary operator and dramatically reduce the number of quantum gates of the traditional approach. The average fidelity between the states that are produced by applying target and generated unitary on random input states is around 0.997.Keywords
Cite This Article
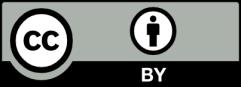
This work is licensed under a Creative Commons Attribution 4.0 International License , which permits unrestricted use, distribution, and reproduction in any medium, provided the original work is properly cited.