Open Access
ARTICLE
Evolutionary GAN–Based Data Augmentation for Cardiac Magnetic Resonance Image
1 School of Computer Science, Chengdu University of Information Technology, Chengdu, 610225, China
2 Images and Spatial Information 2011 Collaborative Innovation Center of Sichuan Province, Chengdu, 610225, China
3 Department of Computer Science, University of Reading, Earley, RG6 6AY, UK
* Corresponding Author: Ying Fu. Email:
Computers, Materials & Continua 2021, 68(1), 1359-1374. https://doi.org/10.32604/cmc.2021.016536
Received 04 January 2021; Accepted 09 February 2021; Issue published 22 March 2021
Abstract
Generative adversarial networks (GANs) have considerable potential to alleviate challenges linked to data scarcity. Recent research has demonstrated the good performance of this method for data augmentation because GANs synthesize semantically meaningful data from standard signal distribution. The goal of this study was to solve the overfitting problem that is caused by the training process of convolution networks with a small dataset. In this context, we propose a data augmentation method based on an evolutionary generative adversarial network for cardiac magnetic resonance images to extend the training data. In our structure of the evolutionary GAN, the most optimal generator is chosen that considers the quality and diversity of generated images simultaneously from many generator mutations. Also, to expand the distribution of the whole training set, we combine the linear interpolation of eigenvectors to synthesize new training samples and synthesize related linear interpolation labels. This approach makes the discrete sample space become continuous and improves the smoothness between domains. The visual quality of the augmented cardiac magnetic resonance images is improved by our proposed method as shown by the data-augmented experiments. In addition, the effectiveness of our proposed method is verified by the classification experiments. The influence of the proportion of synthesized samples on the classification results of cardiac magnetic resonance images is also explored.Keywords
Cite This Article
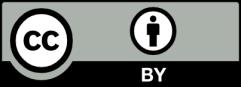
This work is licensed under a Creative Commons Attribution 4.0 International License , which permits unrestricted use, distribution, and reproduction in any medium, provided the original work is properly cited.