Open Access
ARTICLE
Traffic Engineering in Dynamic Hybrid Segment Routing Networks
1 College of Mathematics and Computer Science, Fuzhou University, Fuzhou, 350000, China
2 Fujian Key Laboratory of Network Computing and Intelligent Information Processing, Fuzhou University, Fuzhou, 350000, China
3 Key Laboratory of Spatial Data Mining & Information Sharing, Ministry of Education, Fuzhou, 350003, China
4 School of Information Science and Technology, Guangdong University of Foreign Studies, Guangzhou, 510006, China
5 School of Computer Science and Cyberspace Security, Hainan University, HaiKou, 570228, China
6 Tandon School of Engineering, New York University, New York, 10012, USA
7 Department of Computing, Hong Kong Polytechnic University, Hong Hom, 999077, Hong Kong
* Corresponding Author: Cheng Hu. Email:
Computers, Materials & Continua 2021, 68(1), 655-670. https://doi.org/10.32604/cmc.2021.016364
Received 31 December 2020; Accepted 02 February 2021; Issue published 22 March 2021
Abstract
The emergence of Segment Routing (SR) provides a novel routing paradigm that uses a routing technique called source packet routing. In SR architecture, the paths that the packets choose to route on are indicated at the ingress router. Compared with shortest-path-based routing in traditional distributed routing protocols, SR can realize a flexible routing by implementing an arbitrary flow splitting at the ingress router. Despite the advantages of SR, it may be difficult to update the existing IP network to a full SR deployed network, for economical and technical reasons. Updating partial of the traditional IP network to the SR network, thus forming a hybrid SR network, is a preferable choice. For the traffic is dynamically changing in a daily time, in this paper, we propose a Weight Adjustment algorithm WASAR to optimize routing in a dynamic hybrid SR network. WASAR algorithm can be divided into three steps: firstly, representative Traffic Matrices (TMs) and the expected TM are obtained from the historical TMs through ultra-scalable spectral clustering algorithm. Secondly, given the network topology, the initial network weight setting and the expected TM, we can realize the link weight optimization and SR node deployment optimization through a Deep Reinforcement Learning (DRL) algorithm. Thirdly, we optimize the flow splitting ratios of SR nodes in a centralized online manner under dynamic traffic demands, in order to improve the network performance. In the evaluation, we exploit historical TMs to test the performance of the obtained routing configuration in WASAR. The extensive experimental results validate that our proposed WASAR algorithm has superior performance in reducing Maximum Link Utilization (MLU) under the dynamic traffic.Keywords
Cite This Article
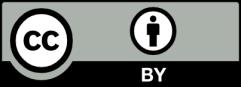
This work is licensed under a Creative Commons Attribution 4.0 International License , which permits unrestricted use, distribution, and reproduction in any medium, provided the original work is properly cited.