Open Access
ARTICLE
Computer Decision Support System for Skin Cancer Localization and Classification
1 Department of Computer Science, COMSATS University Islamabad, Wah Campus, 47040, Pakistan
2 Department of Computer and Electrical Engineering, COMSATS University Islamabad, Wah Campus, 47040, Pakistan
3 Department of Mathematics, Beirut Arab University, Beirut, Lebanon
4 Department of Computer Science and Engineering, Soonchunhyang University, Asan, Korea
* Corresponding Author: Yunyoung Nam. Email:
(This article belongs to the Special Issue: Intelligent Decision Support Systems for Complex Healthcare Applications)
Computers, Materials & Continua 2021, 68(1), 1041-1064. https://doi.org/10.32604/cmc.2021.016307
Received 30 December 2020; Accepted 02 February 2021; Issue published 22 March 2021
Abstract
In this work, we propose a new, fully automated system for multiclass skin lesion localization and classification using deep learning. The main challenge is to address the problem of imbalanced data classes, found in HAM10000, ISBI2018, and ISBI2019 datasets. Initially, we consider a pre-trained deep neural network model, DarkeNet19, and fine-tune the parameters of third convolutional layer to generate the image gradients. All the visualized images are fused using a High-Frequency approach along with Multilayered Feed-Forward Neural Network (HFaFFNN). The resultant image is further enhanced by employing a log-opening based activation function to generate a localized binary image. Later, two pre-trained deep models, Darknet-53 and NasNet-mobile, are employed and fine-tuned according to the selected datasets. The concept of transfer learning is later explored to train both models, where the input feed is the generated localized lesion images. In the subsequent step, the extracted features are fused using parallel max entropy correlation (PMEC) technique. To avoid the problem of overfitting and to select the most discriminant feature information, we implement a hybrid optimization algorithm called entropy-kurtosis controlled whale optimization (EKWO) algorithm. The selected features are finally passed to the softmax classifier for the final classification. Three datasets are used for the experimental process, such as HAM10000, ISBI2018, and ISBI2019 to achieve an accuracy of 95.8%, 97.1%, and 85.35%, respectively.Keywords
Cite This Article
Citations
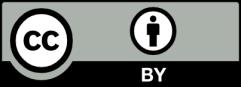
This work is licensed under a Creative Commons Attribution 4.0 International License , which permits unrestricted use, distribution, and reproduction in any medium, provided the original work is properly cited.