Open Access
ARTICLE
A Knowledge-Enriched and Span-Based Network for Joint Entity and Relation Extraction
1 The Sixty-Third Research Institute, National University of Defense Technology, Nanjing, 210007, China
2 School of Computer Science and Technology, Southeast University, Nanjing, 211189, China
3 Faculty of Information Technology, Monash University, Melbourne, 3800, Australia
* Corresponding Author: Xiaoxiong Zhang. Email:
Computers, Materials & Continua 2021, 68(1), 377-389. https://doi.org/10.32604/cmc.2021.016301
Received 29 December 2020; Accepted 29 January 2021; Issue published 22 March 2021
Abstract
The joint extraction of entities and their relations from certain texts plays a significant role in most natural language processes. For entity and relation extraction in a specific domain, we propose a hybrid neural framework consisting of two parts: a span-based model and a graph-based model. The span-based model can tackle overlapping problems compared with BILOU methods, whereas the graph-based model treats relation prediction as graph classification. Our main contribution is to incorporate external lexical and syntactic knowledge of a specific domain, such as domain dictionaries and dependency structures from texts, into end-to-end neural models. We conducted extensive experiments on a Chinese military entity and relation extraction corpus. The results show that the proposed framework outperforms the baselines with better performance in terms of entity and relation prediction. The proposed method provides insight into problems with the joint extraction of entities and their relations.Keywords
Cite This Article
Citations
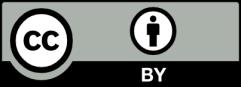
This work is licensed under a Creative Commons Attribution 4.0 International License , which permits unrestricted use, distribution, and reproduction in any medium, provided the original work is properly cited.