Open Access
ARTICLE
Imperative Dynamic Routing Between Capsules Network for Malaria Classification
1 Department of Information Technology, VNRVJIET, Hyderabad, 500090, India
2 Department of Computer Science and Engineering, JNTUH College of Engineering, Hyderabad, India
3 Department of Computer Science and Engineering, TKR College of Engineering & Technology, Hyderabad, India
4 Department of Computer Science and Engineering, SRM University, Amaravati, A.P., 522502, India
5 School of Computer Science and Engineering, Taylor’s University, Subang Jaya, 47500, Malaysia
6 Department of Computer Science and Engineering, VNRVJIET, Hyderabad, 500090, India
* Corresponding Author: G. Madhu. Email:
Computers, Materials & Continua 2021, 68(1), 903-919. https://doi.org/10.32604/cmc.2021.016114
Received 10 December 2020; Accepted 13 January 2021; Issue published 22 March 2021
Abstract
Malaria is a severe epidemic disease caused by Plasmodium falciparum. The parasite causes critical illness if persisted for longer durations and delay in precise treatment can lead to further complications. The automatic diagnostic model provides aid for medical practitioners to avail a fast and efficient diagnosis. Most of the existing work either utilizes a fully connected convolution neural network with successive pooling layers which causes loss of information in pixels. Further, convolutions can capture spatial invariances but, cannot capture rotational invariances. Hence to overcome these limitations, this research, develops an Imperative Dynamic routing mechanism with fully trained capsule networks for malaria classification. This model identifies the presence of malaria parasites by classifying thin blood smears containing samples of parasitized and healthy erythrocytes. The proposed model is compared and evaluated with novel machine vision models evolved over a decade such as VGG, ResNet, DenseNet, MobileNet. The problems in previous research are cautiously addressed and overhauled using the proposed capsule network by attaining the highest Area under the curve (AUC) and Specificity of 99.03% and 99.43% respectively for 20% test samples. To understand the underlying behavior of the proposed network various tests are conducted for variant shuffle patterns. The model is analyzed and assessed in distinct environments to depict its resilience and versatility. To provide greater generalization, the proposed network has been tested on thick blood smear images which surpassed with greater performance.Keywords
Cite This Article
Citations
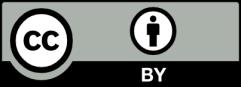