Open Access
ARTICLE
COVID-19 Infected Lung Computed Tomography Segmentation and Supervised Classification Approach
1 Department of Computer Science, Concordia College Bahawalpur, Bahawalpur, 63100, Pakistan
2 Department of Computer Science & IT, Glim Institute of Modern Studies, Bahawalpur, 63100, Pakistan
3 Institute of Numerical Sciences, Kohat University of Science & Technology, Kohat, 26000, Pakistan
4 Institute of Computing, Kohat University of Science and Technology, Kohat, 26000, Pakistan
5 Program in Applied Statistics, Department of Mathematics and Computer Science, Faculty of Science and Technology, Rajamangala University of Technology Thanyaburi, Thanyaburi, 12110, Thailand
6 Departments of Mathematics, Faculty of Science, Center of Excellence in Theoretical and Computational Science (TaCS-CoE) & KMUTT Fixed Point Research Laboratory, Room SCL 802 Fixed Point Laboratory, Science Laboratory Building, King Mongkut’s University of Technology Thonburi (KMUTT), Bangkok, 10140, Thailand
7 Department of Medical Research, China Medical University Hospital, Taichung, 40402, Taiwan
8 College of Engineering, Al Ain University, Al Ain, 64141, United Arab Emirates
9 Department of Mathematics, Tafila Technical University, Tafila, 66110, Jordan
10 Department of Statistics, The Islamia University of Bahawalpur, Bahawalpur, 63100, Pakistan
11 Department of Mathematics, Université de Caen, LMNO, Caen, 14032, France
* Corresponding Author: Poom Kumam. Email:
(This article belongs to the Special Issue: Deep Learning and Parallel Computing for Intelligent and Efficient IoT)
Computers, Materials & Continua 2021, 68(1), 391-407. https://doi.org/10.32604/cmc.2021.016037
Received 19 December 2020; Accepted 19 January 2021; Issue published 22 March 2021
Abstract
The purpose of this research is the segmentation of lungs computed tomography (CT) scan for the diagnosis of COVID-19 by using machine learning methods. Our dataset contains data from patients who are prone to the epidemic. It contains three types of lungs CT images (Normal, Pneumonia, and COVID-19) collected from two different sources; the first one is the Radiology Department of Nishtar Hospital Multan and Civil Hospital Bahawalpur, Pakistan, and the second one is a publicly free available medical imaging database known as Radiopaedia. For the preprocessing, a novel fuzzy c-mean automated region-growing segmentation approach is deployed to take an automated region of interest (ROIs) and acquire 52 hybrid statistical features for each ROIs. Also, 12 optimized statistical features are selected via the chi-square feature reduction technique. For the classification, five machine learning classifiers named as deep learning J4, multilayer perceptron, support vector machine, random forest, and naive Bayes are deployed to optimize the hybrid statistical features dataset. It is observed that the deep learning J4 has promising results (sensitivity and specificity: 0.987; accuracy: 98.67%) among all the deployed classifiers. As a complementary study, a statistical work is devoted to the use of a new statistical model to fit the main datasets of COVID-19 collected in Pakistan.Keywords
Cite This Article
Citations
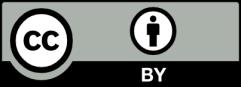
This work is licensed under a Creative Commons Attribution 4.0 International License , which permits unrestricted use, distribution, and reproduction in any medium, provided the original work is properly cited.