Open Access
ARTICLE
Deep Learning Enabled Autoencoder Architecture for Collaborative Filtering Recommendation in IoT Environment
College of Computer Engineering and Sciences, Prince Sattam bin Abdulaziz University, AlKharj, Saudi Arabia
* Corresponding Author: Thavavel Vaiyapuri. Email:
(This article belongs to the Special Issue: Deep Learning Trends in Intelligent Systems)
Computers, Materials & Continua 2021, 68(1), 487-503. https://doi.org/10.32604/cmc.2021.015998
Received 17 December 2020; Accepted 26 January 2021; Issue published 22 March 2021
Abstract
The era of the Internet of things (IoT) has marked a continued exploration of applications and services that can make people’s lives more convenient than ever before. However, the exploration of IoT services also means that people face unprecedented difficulties in spontaneously selecting the most appropriate services. Thus, there is a paramount need for a recommendation system that can help improve the experience of the users of IoT services to ensure the best quality of service. Most of the existing techniques—including collaborative filtering (CF), which is most widely adopted when building recommendation systems—suffer from rating sparsity and cold-start problems, preventing them from providing high quality recommendations. Inspired by the great success of deep learning in a wide range of fields, this work introduces a deep-learning-enabled autoencoder architecture to overcome the setbacks of CF recommendations. The proposed deep learning model is designed as a hybrid architecture with three key networks, namely autoencoder (AE), multilayered perceptron (MLP), and generalized matrix factorization (GMF). The model employs two AE networks to learn deep latent feature representations of users and items respectively and in parallel. Next, MLP and GMF networks are employed to model the linear and non-linear user-item interactions respectively with the extracted latent user and item features. Finally, the rating prediction is performed based on the idea of ensemble learning by fusing the output of the GMF and MLP networks. We conducted extensive experiments on two benchmark datasets, MoiveLens100K and MovieLens1M, using four standard evaluation metrics. Ablation experiments were conducted to confirm the validity of the proposed model and the contribution of each of its components in achieving better recommendation performance. Comparative analyses were also carried out to demonstrate the potential of the proposed model in gaining better accuracy than the existing CF methods with resistance to rating sparsity and cold-start problems.Keywords
Cite This Article
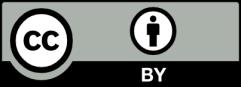
This work is licensed under a Creative Commons Attribution 4.0 International License , which permits unrestricted use, distribution, and reproduction in any medium, provided the original work is properly cited.