Open Access
ARTICLE
Deep Learning Multimodal for Unstructured and Semi-Structured Textual Documents Classification
Faculty of Computers and Information, Department of Computer Science, Mansoura University, 35516, Egypt
* Corresponding Author: Osama Abu-Elnasr. Email:
(This article belongs to the Special Issue: Deep Learning Trends in Intelligent Systems)
Computers, Materials & Continua 2021, 68(1), 589-606. https://doi.org/10.32604/cmc.2021.015761
Received 05 December 2020; Accepted 23 January 2021; Issue published 22 March 2021
Abstract
Due to the availability of a huge number of electronic text documents from a variety of sources representing unstructured and semi-structured information, the document classification task becomes an interesting area for controlling data behavior. This paper presents a document classification multimodal for categorizing textual semi-structured and unstructured documents. The multimodal implements several individual deep learning models such as Deep Neural Networks (DNN), Recurrent Convolutional Neural Networks (RCNN) and Bidirectional-LSTM (Bi-LSTM). The Stacked Ensemble based meta-model technique is used to combine the results of the individual classifiers to produce better results, compared to those reached by any of the above mentioned models individually. A series of textual preprocessing steps are executed to normalize the input corpus followed by text vectorization techniques. These techniques include using Term Frequency Inverse Term Frequency (TFIDF) or Continuous Bag of Word (CBOW) to convert text data into the corresponding suitable numeric form acceptable to be manipulated by deep learning models. Moreover, this proposed model is validated using a dataset collected from several spaces with a huge number of documents in every class. In addition, the experimental results prove that the proposed model has achieved effective performance. Besides, upon investigating the PDF Documents classification, the proposed model has achieved accuracy up to 0.9045 and 0.959 for the TFIDF and CBOW features, respectively. Moreover, concerning the JSON Documents classification, the proposed model has achieved accuracy up to 0.914 and 0.956 for the TFIDF and CBOW features, respectively. Furthermore, as for the XML Documents classification, the proposed model has achieved accuracy values up to 0.92 and 0.959 for the TFIDF and CBOW features, respectively.Keywords
Cite This Article
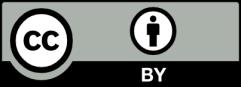
This work is licensed under a Creative Commons Attribution 4.0 International License , which permits unrestricted use, distribution, and reproduction in any medium, provided the original work is properly cited.