Open Access
ARTICLE
Deep Learning-Based Hybrid Intelligent Intrusion Detection System
1 Department of Information, and Communication Engineering, Dongguk University, Seoul, 100-715, Korea
2 Department of Electronics Engineering, IoT and Big-Data Research Center, Incheon National University, Incheon, Korea
* Corresponding Author: Yangwoo Kim. Email:
(This article belongs to the Special Issue: Machine Learning-based Intelligent Systems: Theories, Algorithms, and Applications)
Computers, Materials & Continua 2021, 68(1), 671-687. https://doi.org/10.32604/cmc.2021.015647
Received 01 December 2020; Accepted 24 January 2021; Issue published 22 March 2021
Abstract
Machine learning (ML) algorithms are often used to design effective intrusion detection (ID) systems for appropriate mitigation and effective detection of malicious cyber threats at the host and network levels. However, cybersecurity attacks are still increasing. An ID system can play a vital role in detecting such threats. Existing ID systems are unable to detect malicious threats, primarily because they adopt approaches that are based on traditional ML techniques, which are less concerned with the accurate classification and feature selection. Thus, developing an accurate and intelligent ID system is a priority. The main objective of this study was to develop a hybrid intelligent intrusion detection system (HIIDS) to learn crucial features representation efficiently and automatically from massive unlabeled raw network traffic data. Many ID datasets are publicly available to the cybersecurity research community. As such, we used a spark MLlib (machine learning library)-based robust classifier, such as logistic regression (LR), extreme gradient boosting (XGB) was used for anomaly detection, and a state-of-the-art DL, such as a long short-term memory autoencoder (LSTMAE) for misuse attack was used to develop an efficient and HIIDS to detect and classify unpredictable attacks. Our approach utilized LSTM to detect temporal features and an AE to more efficiently detect global features. Therefore, to evaluate the efficacy of our proposed approach, experiments were conducted on a publicly existing dataset, the contemporary real-life ISCX-UNB dataset. The simulation results demonstrate that our proposed spark MLlib and LSTMAE-based HIIDS significantly outperformed existing ID approaches, achieving a high accuracy rate of up to 97.52% for the ISCX-UNB dataset respectively 10-fold cross-validation test. It is quite promising to use our proposed HIIDS in real-world circumstances on a large-scale.Keywords
Cite This Article
Citations
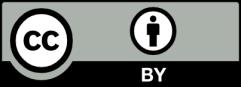
This work is licensed under a Creative Commons Attribution 4.0 International License , which permits unrestricted use, distribution, and reproduction in any medium, provided the original work is properly cited.