Open Access
ARTICLE
Classification of COVID-19 CT Scans via Extreme Learning Machine
1 Department of Computer Science, HITEC University, Taxila, 47040, Pakistan
2 Department of Electrical Engineering, COMSATS University Islamabad, Wah Campus, Wah Cantt, Pakistan
3 Department of Computer Science and Engineering, Soonchunhyang University, Asan, Korea
4 Department of Mathematics and Computer Science, Faculty of Science, Beirut Arab University, Lebanon
5 Department of Mathematics, University of Leicester, Leicester, UK
6 College of Computer Science and Engineering, University of Ha’il, Ha’il, Saudi Arabia
* Corresponding Author: Yunyoung Nam. Email:
(This article belongs to the Special Issue: Artificial Intelligence and IoT based intelligent systems using high performance computing for Medical applications.)
Computers, Materials & Continua 2021, 68(1), 1003-1019. https://doi.org/10.32604/cmc.2021.015541
Received 26 November 2020; Accepted 23 January 2021; Issue published 22 March 2021
Abstract
Here, we use multi-type feature fusion and selection to predict COVID-19 infections on chest computed tomography (CT) scans. The scheme operates in four steps. Initially, we prepared a database containing COVID-19 pneumonia and normal CT scans. These images were retrieved from the Radiopaedia COVID-19 website. The images were divided into training and test sets in a ratio of 70:30. Then, multiple features were extracted from the training data. We used canonical correlation analysis to fuse the features into single vectors; this enhanced the predictive capacity. We next implemented a genetic algorithm (GA) in which an Extreme Learning Machine (ELM) served to assess GA fitness. Based on the ELM losses, the most discriminatory features were selected and saved as an ELM Model. Test images were sent to the model, and the best-selected features compared to those of the trained model to allow final predictions. Validation employed the collected chest CT scans. The best predictive accuracy of the ELM classifier was 93.9%; the scheme was effective.Keywords
Cite This Article
Citations
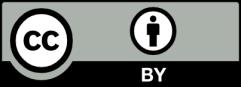
This work is licensed under a Creative Commons Attribution 4.0 International License , which permits unrestricted use, distribution, and reproduction in any medium, provided the original work is properly cited.