Open Access
ARTICLE
An Optimized Deep Residual Network with a Depth Concatenated Block for Handwritten Characters Classification
Department of Information Systems, King Abdulaziz University, Jeddah, 21589, SA
* Corresponding Author: Gibrael Abosamra. Email:
(This article belongs to the Special Issue: Deep Learning Trends in Intelligent Systems)
Computers, Materials & Continua 2021, 68(1), 1-28. https://doi.org/10.32604/cmc.2021.015318
Received 15 November 2020; Accepted 24 December 2020; Issue published 22 March 2021
Abstract
Even though much advancements have been achieved with regards to the recognition of handwritten characters, researchers still face difficulties with the handwritten character recognition problem, especially with the advent of new datasets like the Extended Modified National Institute of Standards and Technology dataset (EMNIST). The EMNIST dataset represents a challenge for both machine-learning and deep-learning techniques due to inter-class similarity and intra-class variability. Inter-class similarity exists because of the similarity between the shapes of certain characters in the dataset. The presence of intra-class variability is mainly due to different shapes written by different writers for the same character. In this research, we have optimized a deep residual network to achieve higher accuracy vs. the published state-of-the-art results. This approach is mainly based on the prebuilt deep residual network model ResNet18, whose architecture has been enhanced by using the optimal number of residual blocks and the optimal size of the receptive field of the first convolutional filter, the replacement of the first max-pooling filter by an average pooling filter, and the addition of a drop-out layer before the fully connected layer. A distinctive modification has been introduced by replacing the final addition layer with a depth concatenation layer, which resulted in a novel deep architecture having higher accuracy vs. the pure residual architecture. Moreover, the dataset images’ sizes have been adjusted to optimize their visibility in the network. Finally, by tuning the training hyperparameters and using rotation and shear augmentations, the proposed model outperformed the state-of-the-art models by achieving average accuracies of 95.91% and 90.90% for the Letters and Balanced dataset sections, respectively. Furthermore, the average accuracies were improved to 95.9% and 91.06% for the Letters and Balanced sections, respectively, by using a group of 5 instances of the trained models and averaging the output class probabilities.Keywords
Cite This Article
Citations
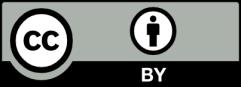