Open Access
ARTICLE
Deep Trajectory Classification Model for Congestion Detection in Human Crowds
1 Department of Computer Engineering, College of Computing and Information Systems, Umm Al-Qura University, Makkah, Saudi Arabia
2 Department of Computer Science, National University of Technology, Islamabad, Pakistan
3 Science and Technology Unit, Umm Al-Qura University, Makkah, Saudi Arabia
4 Institute of Consulting Research and Studies, Umm Al-Qura University, Makkah, Saudi Arabia
* Corresponding Author: Faizan Ur Rehman. Email:
Computers, Materials & Continua 2021, 68(1), 705-725. https://doi.org/10.32604/cmc.2021.015085
Received 06 November 2020; Accepted 02 February 2021; Issue published 22 March 2021
Abstract
In high-density gatherings, crowd disasters frequently occur despite all the safety measures. Timely detection of congestion in human crowds using automated analysis of video footage can prevent crowd disasters. Recent work on the prevention of crowd disasters has been based on manual analysis of video footage. Some methods also measure crowd congestion by estimating crowd density. However, crowd density alone cannot provide reliable information about congestion. This paper proposes a deep learning framework for automated crowd congestion detection that leverages pedestrian trajectories. The proposed framework divided the input video into several temporal segments. We then extracted dense trajectories from each temporal segment and converted these into a spatio-temporal image without losing information. A classification model based on convolutional neural networks was then trained using spatio-temporal images. Next, we generated a score map by encoding each point trajectory with its respective class score. After this, we obtained the congested regions by employing the non-maximum suppression method on the score map. Finally, we demonstrated the proposed framework’s effectiveness by performing a series of experiments on challenging video sequences.Keywords
Cite This Article
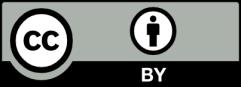
This work is licensed under a Creative Commons Attribution 4.0 International License , which permits unrestricted use, distribution, and reproduction in any medium, provided the original work is properly cited.