Open Access
ARTICLE
A Deep Learning-Based Recognition Approach for the Conversion of Multilingual Braille Images
1 Department of Computer Science, College of Computer and Information Sciences, King Saud University, Riyadh, 11543, Saudi Arabia
2 Department of Special Education, College of Education, King Saud University, Riyadh, 11543, Saudi Arabia
* Corresponding Author: Abdu Gumaei. Email:
Computers, Materials & Continua 2021, 67(3), 3847-3864. https://doi.org/10.32604/cmc.2021.015614
Received 30 November 2020; Accepted 17 January 2021; Issue published 01 March 2021
Abstract
Braille-assistive technologies have helped blind people to write, read, learn, and communicate with sighted individuals for many years. These technologies enable blind people to engage with society and help break down communication barriers in their lives. The Optical Braille Recognition (OBR) system is one example of these technologies. It plays an important role in facilitating communication between sighted and blind people and assists sighted individuals in the reading and understanding of the documents of Braille cells. However, a clear gap exists in current OBR systems regarding asymmetric multilingual conversion of Braille documents. Few systems allow sighted people to read and understand Braille documents for self-learning applications. In this study, we propose a deep learning-based approach to convert Braille images into multilingual texts. This is achieved through a set of effective steps that start with image acquisition and preprocessing and end with a Braille multilingual mapping step. We develop a deep convolutional neural network (DCNN) model that takes its inputs from the second step of the approach for recognizing Braille cells. Several experiments are conducted on two datasets of Braille images to evaluate the performance of the DCNN model. The first dataset contains 1,404 labeled images of 27 Braille symbols representing the alphabet characters. The second dataset consists of 5,420 labeled images of 37 Braille symbols that represent alphabet characters, numbers, and punctuation. The proposed model achieved a classification accuracy of 99.28% on the test set of the first dataset and 98.99% on the test set of the second dataset. These results confirm the applicability of the DCNN model used in our proposed approach for multilingual Braille conversion in communicating with sighted people.Keywords
Cite This Article
Citations
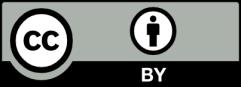