Open Access
ARTICLE
CNN Ensemble Approach to Detect COVID-19 from Computed Tomography Chest Images
Department Computer Engineering, Advanced Lab for Intelligent Systems, College of Computer and Information Sciences, King Saud University, Riyadh, 11543, Saudi Arabia
* Corresponding Author: Haikel Alhichri. Email:
(This article belongs to the Special Issue: Artificial Intelligence and Healthcare Analytics for COVID-19)
Computers, Materials & Continua 2021, 67(3), 3581-3599. https://doi.org/10.32604/cmc.2021.015399
Received 19 November 2020; Accepted 16 January 2021; Issue published 01 March 2021
Abstract
In January 2020, the World Health Organization declared a global health emergency concerning the spread of a new coronavirus disease, which was later named COVID-19. Early and fast diagnosis and isolation of COVID-19 patients have proven to be instrumental in limiting the spread of the disease. Computed tomography (CT) is a promising imaging method for fast diagnosis of COVID-19. In this study, we develop a unique preprocessing step to resize CT chest images to a fixed size (256 × 256 pixels) that preserves the aspect ratio and reduces image loss. Then, we present a deep learning (DL) method to classify CT chest images based on the light-weight pre-trained EfficientNet-B3 CNN model and ensemble techniques. The proposed method, which we refer to as EfficientNet-B3-GAP-Ensemble, comprises an ensemble of a modified version of the EfficientNet-B3. We build the ensemble using multiple runs and multiple training epochs. We test the EfficientNet-B3-GAP-Ensemble on two common benchmark datasets, i.e., the COVID19-CT and SARS-CoV-2-CT datasets. The proposed method has outperformed state-of-the-art methods for both datasets. For the COVID19-CT dataset, it achieved 88.18% sensitivity, 88.29% precision, 88.18% accuracy, an F1-score of 88.15%, and AUC of 92.10%. With the SARS-CoV-2-CT dataset, we tested the proposed method under different train-test splits, i.e., 20%–80%, 50%–50%, and 80%–20%. For the latter split, the proposed method achieved 99.72% accuracy, 99.80% sensitivity, precision, and F1-scores, and an AUC score of 99.99%.Keywords
Cite This Article
Citations
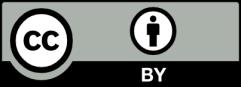