Open Access
ARTICLE
Feasibility-Guided Constraint-Handling Techniques for Engineering Optimization Problems
1 Institute of Numerical Sciences, Kohat University of Science & Technology, Kohat, Pakistan
2 Department of Economics, Abbottabad University of Science & Technology, Abbottabad, Pakistan
3 Institute of Computing, Kohat University of Science & Technology, Kohat, Pakistan
4 Faculty of Applied Studies, King Abdulaziz University, Jeddah, Saudi Arabia
5 Jinnah College for Women, University of Peshawar, Peshawar, Pakistan
6 Department of Physics, Kohat University of Science & Technology, Kohat, Pakistan
* Corresponding Author: Muhammad Asif Jan. Email:
(This article belongs to the Special Issue: Deep Learning and Parallel Computing for Intelligent and Efficient IoT)
Computers, Materials & Continua 2021, 67(3), 2845-2862. https://doi.org/10.32604/cmc.2021.015294
Received 14 November 2020; Accepted 02 January 2021; Issue published 01 March 2021
Abstract
The particle swarm optimization (PSO) algorithm is an established nature-inspired population-based meta-heuristic that replicates the synchronizing movements of birds and fish. PSO is essentially an unconstrained algorithm and requires constraint handling techniques (CHTs) to solve constrained optimization problems (COPs). For this purpose, we integrate two CHTs, the superiority of feasibility (SF) and the violation constraint-handling (VCH), with a PSO. These CHTs distinguish feasible solutions from infeasible ones. Moreover, in SF, the selection of infeasible solutions is based on their degree of constraint violations, whereas in VCH, the number of constraint violations by an infeasible solution is of more importance. Therefore, a PSO is adapted for constrained optimization, yielding two constrained variants, denoted SF-PSO and VCH-PSO. Both SF-PSO and VCH-PSO are evaluated with respect to five engineering problems: the Himmelblau’s nonlinear optimization, the welded beam design, the spring design, the pressure vessel design, and the three-bar truss design. The simulation results show that both algorithms are consistent in terms of their solutions to these problems, including their different available versions. Comparison of the SF-PSO and the VCH-PSO with other existing algorithms on the tested problems shows that the proposed algorithms have lower computational cost in terms of the number of function evaluations used. We also report our disagreement with some unjust comparisons made by other researchers regarding the tested problems and their different variants.Keywords
Cite This Article
M. Asif Jan, Y. Mahmood, H. Ullah Khan, W. Khan Mashwani, M. Irfan Uddin et al., "Feasibility-guided constraint-handling techniques for engineering optimization problems," Computers, Materials & Continua, vol. 67, no.3, pp. 2845–2862, 2021. https://doi.org/10.32604/cmc.2021.015294Citations
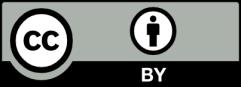