Open Access
ARTICLE
Multiclass Stomach Diseases Classification Using Deep Learning Features Optimization
1 Department of Computer Science, HITEC University, Taxila, 47040, Pakistan
2 College of Computer Science and Engineering, University of Ha’il, Ha’il, Saudi Arabia
3 Department of Informatics, University of Leicester, Leicester, UK
4 Department of Mathematics and Computer Science, Faculty of Science, Beirut Arab University, Lebanon
5 Department of Computer Science and Engineering, Soonchunhyang University, Asan, Korea
* Corresponding Author: Yunyoung Nam. Email:
(This article belongs to the Special Issue: AI, IoT, Blockchain Assisted Intelligent Solutions to Medical and Healthcare Systems)
Computers, Materials & Continua 2021, 67(3), 3381-3399. https://doi.org/10.32604/cmc.2021.014983
Received 31 October 2020; Accepted 16 December 2020; Issue published 01 March 2021
Abstract
In the area of medical image processing, stomach cancer is one of the most important cancers which need to be diagnose at the early stage. In this paper, an optimized deep learning method is presented for multiple stomach disease classification. The proposed method work in few important steps—preprocessing using the fusion of filtering images along with Ant Colony Optimization (ACO), deep transfer learning-based features extraction, optimization of deep extracted features using nature-inspired algorithms, and finally fusion of optimal vectors and classification using Multi-Layered Perceptron Neural Network (MLNN). In the feature extraction step, pre-trained Inception V3 is utilized and retrained on selected stomach infection classes using the deep transfer learning step. Later on, the activation function is applied to Global Average Pool (GAP) for feature extraction. However, the extracted features are optimized through two different nature-inspired algorithms—Particle Swarm Optimization (PSO) with dynamic fitness function and Crow Search Algorithm (CSA). Hence, both methods’ output is fused by a maximal value approach and classified the fused feature vector by MLNN. Two datasets are used to evaluate the proposed method—CUI WahStomach Diseases and Combined dataset and achieved an average accuracy of 99.5%. The comparison with existing techniques, it is shown that the proposed method shows significant performance.Keywords
Cite This Article
Citations
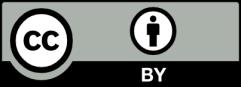
This work is licensed under a Creative Commons Attribution 4.0 International License , which permits unrestricted use, distribution, and reproduction in any medium, provided the original work is properly cited.