Open Access
ARTICLE
Prediction of Cloud Ranking in a Hyperconverged Cloud Ecosystem Using Machine Learning
1 Department of Computer Science, Virtual University of Pakistan, Lahore, 54000, Pakistan
2 Department of Information Sciences, Division of Science & Technology, University of Education, Lahore, 54000, Pakistan
3 Department of Computer Science, Lahore Garrison University, Lahore, 54000, Pakistan
4 School of Computer Science, NCBA&E, Lahore, 54000, Pakistan
5 Department of Computer Science and Information, College of Science in Zulfi, Majmaah University, Al-Majmaah, 11952, Saudi Arabia
6 School of Computer and Information Technology, Beaconhouse National University, Tarogil, Lahore, 53700, Pakistan
7 Faculty of Computing, Riphah School of Computing & Innovation, Riphah International University Lahore Campus, Lahore, 54000, Pakistan
* Corresponding Author: Muhammad Adnan Khan. Email:
Computers, Materials & Continua 2021, 67(3), 3129-3141. https://doi.org/10.32604/cmc.2021.014729
Received 12 October 2020; Accepted 01 January 2021; Issue published 01 March 2021
Abstract
Cloud computing is becoming popular technology due to its functional properties and variety of customer-oriented services over the Internet. The design of reliable and high-quality cloud applications requires a strong Quality of Service QoS parameter metric. In a hyperconverged cloud ecosystem environment, building high-reliability cloud applications is a challenging job. The selection of cloud services is based on the QoS parameters that play essential roles in optimizing and improving cloud rankings. The emergence of cloud computing is significantly reshaping the digital ecosystem, and the numerous services offered by cloud service providers are playing a vital role in this transformation. Hyperconverged software-based unified utilities combine storage virtualization, compute virtualization, and network virtualization. The availability of the latter has also raised the demand for QoS. Due to the diversity of services, the respective quality parameters are also in abundance and need a carefully designed mechanism to compare and identify the critical, common, and impactful parameters. It is also necessary to reconsider the market needs in terms of service requirements and the QoS provided by various CSPs. This research provides a machine learning-based mechanism to monitor the QoS in a hyperconverged environment with three core service parameters: service quality, downtime of servers, and outage of cloud services.Keywords
Cite This Article
N. Tabassum, A. Ditta, T. Alyas, S. Abbas, H. Alquhayz et al., "Prediction of cloud ranking in a hyperconverged cloud ecosystem using machine learning," Computers, Materials & Continua, vol. 67, no.3, pp. 3129–3141, 2021. https://doi.org/10.32604/cmc.2021.014729Citations
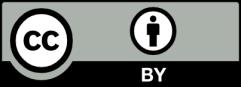