Open Access
ARTICLE
Residual U-Network for Breast Tumor Segmentation from Magnetic Resonance Images
1 Department of Computer Science & Engineering, Bharati Vidyapeeth’s College of Engineering, New Delhi, 110063, India
2 Department of Computer Science & Engineering, G B Pant Govt. College of Engineering, New Delhi, 110020, India
3 Glocal Campus, Konkuk University, Chungju-si Chungcheongbuk-do, 27478, Korea
4 Washington University in St. Louis, MO, 63110, USA
* Corresponding Author: Tai-hoon Kim. Email:
(This article belongs to the Special Issue: Deep Learning Trends in Intelligent Systems)
Computers, Materials & Continua 2021, 67(3), 3107-3127. https://doi.org/10.32604/cmc.2021.014229
Received 07 September 2020; Accepted 30 November 2020; Issue published 01 March 2021
Abstract
Breast cancer positions as the most well-known threat and the main source of malignant growth-related morbidity and mortality throughout the world. It is apical of all new cancer incidences analyzed among females. Two features substantially influence the classification accuracy of malignancy and benignity in automated cancer diagnostics. These are the precision of tumor segmentation and appropriateness of extracted attributes required for the diagnosis. In this research, the authors have proposed a ResU-Net (Residual U-Network) model for breast tumor segmentation. The proposed methodology renders augmented, and precise identification of tumor regions and produces accurate breast tumor segmentation in contrast-enhanced MR images. Furthermore, the proposed framework also encompasses the residual network technique, which subsequently enhances the performance and displays the improved training process. Over and above, the performance of ResU-Net has experimentally been analyzed with conventional U-Net, FCN8, FCN32. Algorithm performance is evaluated in the form of dice coefficient and MIoU (Mean Intersection of Union), accuracy, loss, sensitivity, specificity, F1score. Experimental results show that ResU-Net achieved validation accuracy & dice coefficient value of 73.22% & 85.32% respectively on the Rider Breast MRI dataset and outperformed as compared to the other algorithms used in experimentation.Keywords
Cite This Article
Citations
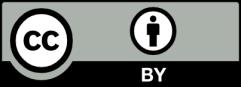