Open Access
ARTICLE
Improving Language Translation Using the Hidden Markov Model
1 School of computer science and engineering, Hunan University of Science and Technology, Xiang, 411201, China
2 School of Info Technology, Deakin University, Geelong, 3220, Australia
* Corresponding Author: Xiaoliang Wang. Email:
Computers, Materials & Continua 2021, 67(3), 3921-3931. https://doi.org/10.32604/cmc.2021.012304
Received 30 August 2020; Accepted 30 December 2020; Issue published 01 March 2021
Abstract
Translation software has become an important tool for communication between different languages. People’s requirements for translation are higher and higher, mainly reflected in people’s desire for barrier free cultural exchange. With a large corpus, the performance of statistical machine translation based on words and phrases is limited due to the small size of modeling units. Previous statistical methods rely primarily on the size of corpus and number of its statistical results to avoid ambiguity in translation, ignoring context. To support the ongoing improvement of translation methods built upon deep learning, we propose a translation algorithm based on the Hidden Markov Model to improve the use of context in the process of translation. During translation, our Hidden Markov Model prediction chain selects a number of phrases with the highest result probability to form a sentence. The collection of all of the generated sentences forms a topic sequence. Using probabilities and article sequences determined from the training set, our method again applies the Hidden Markov Model to form the final translation to improve the context relevance in the process of translation. This algorithm improves the accuracy of translation, avoids the combination of invalid words, and enhances the readability and meaning of the resulting translation.Keywords
Cite This Article
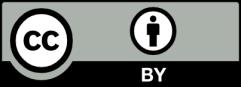
This work is licensed under a Creative Commons Attribution 4.0 International License , which permits unrestricted use, distribution, and reproduction in any medium, provided the original work is properly cited.