Open Access
ARTICLE
Deep Learning Approach for COVID-19 Detection in Computed Tomography Images
1 Department of Applied Computer Science, College of Applied Computer Engineering, King Saud University, Riyadh, 11362, Saudi Arabia
2 Department of Computer Engineering, College of Computer and Information Science, King Saud University, Riyadh, 11362, Saudi Arabia
3 Department of Computer Science, College of Computer and Information Science, King Saud University, Riyadh, 11362, Saudi Arabia
* Corresponding Author: Yakoub Bazi. Email:
(This article belongs to the Special Issue: Artificial Intelligence and Healthcare Analytics for COVID-19)
Computers, Materials & Continua 2021, 67(2), 2093-2110. https://doi.org/10.32604/cmc.2021.014956
Received 29 October 2020; Accepted 13 December 2020; Issue published 05 February 2021
Abstract
With the rapid spread of the coronavirus disease 2019 (COVID-19) worldwide, the establishment of an accurate and fast process to diagnose the disease is important. The routine real-time reverse transcription-polymerase chain reaction (rRT-PCR) test that is currently used does not provide such high accuracy or speed in the screening process. Among the good choices for an accurate and fast test to screen COVID-19 are deep learning techniques. In this study, a new convolutional neural network (CNN) framework for COVID-19 detection using computed tomography (CT) images is proposed. The EfficientNet architecture is applied as the backbone structure of the proposed network, in which feature maps with different scales are extracted from the input CT scan images. In addition, atrous convolution at different rates is applied to these multi-scale feature maps to generate denser features, which facilitates in obtaining COVID-19 findings in CT scan images. The proposed framework is also evaluated in this study using a public CT dataset containing 2482 CT scan images from patients of both classes (i.e., COVID-19 and non-COVID-19). To augment the dataset using additional training examples, adversarial examples generation is performed. The proposed system validates its superiority over the state-of-the-art methods with values exceeding 99.10% in terms of several metrics, such as accuracy, precision, recall, and F1. The proposed system also exhibits good robustness, when it is trained using a small portion of data (20%), with an accuracy of 96.16%.Keywords
Cite This Article
Citations
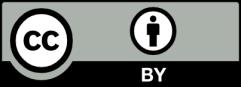