Open Access
ARTICLE
PeachNet: Peach Diseases Detection for Automatic Harvesting
1 Department of Information Technology, College of Computers and Information Technology, Taif University, Taif, 21944, Saudi Arabia
2 Department of Computer Science, College of Computers and Information Technology, Taif University, Taif, 21944, Saudi Arabia
3 Institute of Computing, Kohat University of Science and Technology, Kohat, 26000, Pakistan
* Corresponding Author: Wael Alosaimi. Email:
(This article belongs to the Special Issue: Deep Learning and Parallel Computing for Intelligent and Efficient IoT)
Computers, Materials & Continua 2021, 67(2), 1665-1677. https://doi.org/10.32604/cmc.2021.014950
Received 29 October 2020; Accepted 26 November 2020; Issue published 05 February 2021
Abstract
To meet the food requirements of the seven billion people on Earth, multiple advancements in agriculture and industry have been made. The main threat to food items is from diseases and pests which affect the quality and quantity of food. Different scientific mechanisms have been developed to protect plants and fruits from pests and diseases and to increase the quantity and quality of food. Still these mechanisms require manual efforts and human expertise to diagnose diseases. In the current decade Artificial Intelligence is used to automate different processes, including agricultural processes, such as automatic harvesting. Machine Learning techniques are becoming popular to process images and identify different objects. We can use Machine Learning algorithms for disease identification in plants for automatic harvesting that can help us to increase the quantity of the food produced and reduce crop losses. In this paper, we develop a novel Convolutional Neural Network (CNN) model that can detect diseases in peach plants and fruits. The proposed method can also locate the region of disease and help farmers to find appropriate treatments to protect peach crops. For the detection of diseases in Peaches VGG-19 architecture is utilized. For the localization of disease regions Mask R-CNN is utilized. The proposed technique is evaluated using different techniques and has demonstrated 94% accuracy. We hope that the system can help farmers to increase peach production to meet food demands.Keywords
Cite This Article
Citations
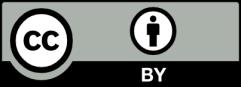
This work is licensed under a Creative Commons Attribution 4.0 International License , which permits unrestricted use, distribution, and reproduction in any medium, provided the original work is properly cited.