Open Access
ARTICLE
Estimation Performance for the Cubature Particle Filter under Nonlinear/Non-Gaussian Environments
1 Department of Communications, Navigation and Control Engineering, National Taiwan Ocean University, Keelung, 202301, Taiwan
2 National Center for High-Performance Computing, National Applied Research Laboratories, Hsinchu, 30076, Taiwan
* Corresponding Author: Dah-Jing Jwo. Email:
Computers, Materials & Continua 2021, 67(2), 1555-1575. https://doi.org/10.32604/cmc.2021.014875
Received 23 October 2020; Accepted 12 December 2020; Issue published 05 February 2021
Abstract
This paper evaluates the state estimation performance for processing nonlinear/non-Gaussian systems using the cubature particle filter (CPF), which is an estimation algorithm that combines the cubature Kalman filter (CKF) and the particle filter (PF). The CPF is essentially a realization of PF where the third-degree cubature rule based on numerical integration method is adopted to approximate the proposal distribution. It is beneficial where the CKF is used to generate the importance density function in the PF framework for effectively resolving the nonlinear/non-Gaussian problems. Based on the spherical-radial transformation to generate an even number of equally weighted cubature points, the CKF uses cubature points with the same weights through the spherical-radial integration rule and employs an analytical probability density function (pdf) to capture the mean and covariance of the posterior distribution using the total probability theorem and subsequently uses the measurement to update with Bayes’ rule. It is capable of acquiring a maximum a posteriori probability estimate of the nonlinear system, and thus the importance density function can be used to approximate the true posterior density distribution. In Bayesian filtering, the nonlinear filter performs well when all conditional densities are assumed Gaussian. When applied to the nonlinear/non-Gaussian distribution systems, the CPF algorithm can remarkably improve the estimation accuracy as compared to the other particle filter-based approaches, such as the extended particle filter (EPF), and unscented particle filter (UPF), and also the Kalman filter (KF)-type approaches, such as the extended Kalman filter (EKF), unscented Kalman filter (UKF) and CKF. Two illustrative examples are presented showing that the CPF achieves better performance as compared to the other approaches.Keywords
Cite This Article
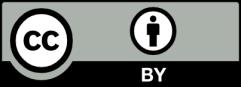
This work is licensed under a Creative Commons Attribution 4.0 International License , which permits unrestricted use, distribution, and reproduction in any medium, provided the original work is properly cited.