Open Access
ARTICLE
A Machine Learning Approach for Expression Detection in Healthcare Monitoring Systems
1 Department of Computer Science & Software Engineering, International Islamic University, Islamabad, 44000, Pakistan
2 Department of Computer Science, Quaid-i-Azam University, Islamabad, 44000, Pakistan
3 Department of Computer Science, Capital University of Science & Technology, Islamabad, 44000, Pakistan
4 Department of Software Engineering, Foundation University, Islamabad, 44000, Pakistan
5 Department of Computer Science, Abdul Wali Khan University, Mardan, 23200, Pakistan
6 Department of Computer Science and Information Systems, College of Business Studies, PAAET, 12062, Kuwait
7 Department of Software, Sejong University, Seoul, 05006, Korea
* Corresponding Author: Oh-Young Song. Email:
Computers, Materials & Continua 2021, 67(2), 2123-2139. https://doi.org/10.32604/cmc.2021.014782
Received 16 October 2020; Accepted 13 December 2020; Issue published 05 February 2021
Abstract
Expression detection plays a vital role to determine the patient’s condition in healthcare systems. It helps the monitoring teams to respond swiftly in case of emergency. Due to the lack of suitable methods, results are often compromised in an unconstrained environment because of pose, scale, occlusion and illumination variations in the image of the face of the patient. A novel patch-based multiple local binary patterns (LBP) feature extraction technique is proposed for analyzing human behavior using facial expression recognition. It consists of three-patch [TPLBP] and four-patch LBPs [FPLBP] based feature engineering respectively. Image representation is encoded from local patch statistics using these descriptors. TPLBP and FPLBP capture information that is encoded to find likenesses between adjacent patches of pixels by using short bit strings contrary to pixel-based methods. Coded images are transformed into the frequency domain using a discrete cosine transform (DCT). Most discriminant features extracted from coded DCT images are combined to generate a feature vector. Support vector machine (SVM), k-nearest neighbor (KNN), and Naïve Bayes (NB) are used for the classification of facial expressions using selected features. Extensive experimentation is performed to analyze human behavior by considering standard extended Cohn Kanade (CK+) and Oulu–CASIA datasets. Results demonstrate that the proposed methodology outperforms the other techniques used for comparison.Keywords
Cite This Article
M. Kashif, A. Hussain, A. Munir, A. Basit Siddiqui, A. Afzaal Abbasi et al., "A machine learning approach for expression detection in healthcare monitoring systems," Computers, Materials & Continua, vol. 67, no.2, pp. 2123–2139, 2021. https://doi.org/10.32604/cmc.2021.014782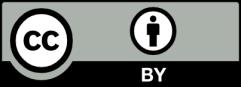