Open Access
ARTICLE
Optimized Deep Learning-Inspired Model for the Diagnosis and Prediction of COVID-19
1 MISR Higher Institute for Engineering and Technology, Mansoura, Egypt
2 Faculty of Computers and Information, Cairo University, Cairo, Egypt
3 VSB-Technical University of Ostrava, Czech Republic
* Corresponding Author: Aboul Ella Hassnien. Email:
Computers, Materials & Continua 2021, 67(2), 2353-2371. https://doi.org/10.32604/cmc.2021.014767
Received 14 October 2020; Accepted 12 December 2020; Issue published 05 February 2021
Abstract
Detecting COVID-19 cases as early as possible became a critical issue that must be addressed to avoid the pandemic’s additional spread and early provide the appropriate treatment to the affected patients. This study aimed to develop a COVID-19 diagnosis and prediction (AIMDP) model that could identify patients with COVID-19 and distinguish it from other viral pneumonia signs detected in chest computed tomography (CT) scans. The proposed system uses convolutional neural networks (CNNs) as a deep learning technology to process hundreds of CT chest scan images and speeds up COVID-19 case prediction to facilitate its containment. We employed the whale optimization algorithm (WOA) to select the most relevant patient signs. A set of experiments validated AIMDP performance. It demonstrated the superiority of AIMDP in terms of the area under the curve-receiver operating characteristic (AUC-ROC) curve, positive predictive value (PPV), negative predictive rate (NPR) and negative predictive value (NPV). AIMDP was applied to a dataset of hundreds of real data and CT images, and it was found to achieve 96% AUC for diagnosing COVID-19 and 98% for overall accuracy. The results showed the promising performance of AIMDP for diagnosing COVID-19 when compared to other recent diagnosing and predicting models.Keywords
Cite This Article
Citations
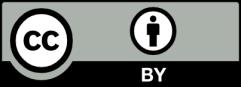