Open Access
ARTICLE
Recognition and Detection of Diabetic Retinopathy Using Densenet-65 Based Faster-RCNN
1 Department of Information Technology, College of Computer, Qassim University, Buraydah, Saudi Arabia
2 Department of Computer Science, University of Engineering and Technology, Taxila, 47050, Pakistan
3 Department of Software Engineering, University of Engineering and Technology, Taxila, 47050, Pakistan
* Corresponding Author: Tahira Nazir. Email:
(This article belongs to the Special Issue: Machine Learning-based Intelligent Systems: Theories, Algorithms, and Applications)
Computers, Materials & Continua 2021, 67(2), 1333-1351. https://doi.org/10.32604/cmc.2021.014691
Received 09 October 2020; Accepted 22 November 2020; Issue published 05 February 2021
Abstract
Diabetes is a metabolic disorder that results in a retinal complication called diabetic retinopathy (DR) which is one of the four main reasons for sightlessness all over the globe. DR usually has no clear symptoms before the onset, thus making disease identification a challenging task. The healthcare industry may face unfavorable consequences if the gap in identifying DR is not filled with effective automation. Thus, our objective is to develop an automatic and cost-effective method for classifying DR samples. In this work, we present a custom Faster-RCNN technique for the recognition and classification of DR lesions from retinal images. After pre-processing, we generate the annotations of the dataset which is required for model training. Then, introduce DenseNet-65 at the feature extraction level of Faster-RCNN to compute the representative set of key points. Finally, the Faster-RCNN localizes and classifies the input sample into five classes. Rigorous experiments performed on a Kaggle dataset comprising of 88,704 images show that the introduced methodology outperforms with an accuracy of 97.2%. We have compared our technique with state-of-the-art approaches to show its robustness in term of DR localization and classification. Additionally, we performed cross-dataset validation on the Kaggle and APTOS datasets and achieved remarkable results on both training and testing phases.Keywords
Cite This Article
Citations
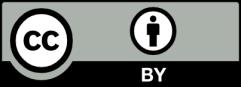
This work is licensed under a Creative Commons Attribution 4.0 International License , which permits unrestricted use, distribution, and reproduction in any medium, provided the original work is properly cited.