Open Access
ARTICLE
Deep Learning and Holt-Trend Algorithms for Predicting Covid-19 Pandemic
1 Community College of Abqaiq, King Faisal University, Al Hofuf, Saudi Arabia
2 Department of Quantitative Methods, School of Business, King Faisal University, Al Hofuf, Saudi Arabia
3 Deanship of E-Learning and Distance Education, King Faisal University, Al Hofuf, Saudi Arabia
4 Department of Computer Sciences and Information Technology, Albaha University, Al Bahah, Saudi Arabia
5 Department of Computer Science and Information, Taibah University, Madinah, Kingdom of Saudi Arabia
* Corresponding Author: Theyazn H. H. Aldhyani. Email:
(This article belongs to the Special Issue: Mathematical aspects of the Coronavirus Disease 2019 (COVID-19): Analysis and Control)
Computers, Materials & Continua 2021, 67(2), 2141-2160. https://doi.org/10.32604/cmc.2021.014498
Received 24 September 2020; Accepted 22 November 2020; Issue published 05 February 2021
Abstract
The Covid-19 epidemic poses a serious public health threat to the world, where people with little or no pre-existing human immunity can be more vulnerable to its effects. Thus, developing surveillance systems for predicting the Covid-19 pandemic at an early stage could save millions of lives. In this study, a deep learning algorithm and a Holt-trend model are proposed to predict the coronavirus. The Long-Short Term Memory (LSTM) and Holt-trend algorithms were applied to predict confirmed numbers and death cases. The real time data used has been collected from the World Health Organization (WHO). In the proposed research, we have considered three countries to test the proposed model, namely Saudi Arabia, Spain and Italy. The results suggest that the LSTM models show better performance in predicting the cases of coronavirus patients. Standard measure performance Mean squared Error (MSE), Root Mean Squared Error (RMSE), Mean error and correlation are employed to estimate the results of the proposed models. The empirical results of the LSTM, using the correlation metrics, are 99.94%, 99.94% and 99.91% in predicting the number of confirmed cases in the three countries. As far as the results of the LSTM model in predicting the number of death of Covid-19, they are 99.86%, 98.876% and 99.16% with respect to Saudi Arabia, Italy and Spain respectively. Similarly, the experiment’s results of the Holt-Trend model in predicting the number of confirmed cases of Covid-19, using the correlation metrics, are 99.06%, 99.96% and 99.94%, whereas the results of the Holt-Trend model in predicting the number of death cases are 99.80%, 99.96% and 99.94% with respect to the Saudi Arabia, Italy and Spain respectively. The empirical results indicate the efficient performance of the presented model in predicting the number of confirmed and death cases of Covid-19 in these countries. Such findings provide better insights regarding the future of Covid-19 this pandemic in general. The results were obtained by applying time series models, which need to be considered for the sake of saving the lives of many people.Keywords
Cite This Article
T. H. H. Aldhyani, M. Alrasheed, M. Hmoud Al-Adaileh, A. Abdullah Alqarni, M. Y. Alzahrani et al., "Deep learning and holt-trend algorithms for predicting covid-19 pandemic," Computers, Materials & Continua, vol. 67, no.2, pp. 2141–2160, 2021. https://doi.org/10.32604/cmc.2021.014498Citations
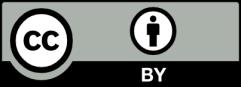