Open Access
ARTICLE
Machine Learning-Enabled Power Scheduling in IoT-Based Smart Cities
1 Department of Computer Science, Government Girls Degree College Mardan, KPK, 23200, Pakistan
2 Department of Electrical Energy System, UET, Peshawar, 25000, Pakistan
3 Department of Computer Science, College of Computing and Informatics, Saudi Electronic University, KSA
4 Department of Smart Computing, Kyungdong University, Global Campus, Gosung, 24764, South Korea
5 Department of Information Sciences, College of Computer and Information Systems, Umm Al-Qura University, Makkah, Saudi Arabia
6 Robotics Engineering, Daegu Gyeongbuk Institute of Science and Technology, South Korea
* Corresponding Author: Nur Alam MD. Email:
(This article belongs to the Special Issue: Machine Learning-based Secured and Privacy-preserved Smart City)
Computers, Materials & Continua 2021, 67(2), 2449-2462. https://doi.org/10.32604/cmc.2021.014386
Received 17 September 2020; Accepted 29 November 2020; Issue published 05 February 2021
Abstract
Recent advancements in hardware and communication technologies have enabled worldwide interconnection using the internet of things (IoT). The IoT is the backbone of smart city applications such as smart grids and green energy management. In smart cities, the IoT devices are used for linking power, price, energy, and demand information for smart homes and home energy management (HEM) in the smart grids. In complex smart grid-connected systems, power scheduling and secure dispatch of information are the main research challenge. These challenges can be resolved through various machine learning techniques and data analytics. In this paper, we have proposed a particle swarm optimization based machine learning algorithm known as a collaborative execute-before-after dependency-based requirement, for the smart grid. The proposed collaborative execute-before-after dependency-based requirement algorithm works in two phases, analysis and assessment of the requirements of end-users and power distribution companies. In the first phases, a fixed load is adjusted over a period of 24 h, and in the second phase, a randomly produced population load for 90 days is evaluated using particle swarm optimization. The simulation results demonstrate that the proposed algorithm performed better in terms of percentage cost reduction, peak to average ratio, and power variance mean ratio than particle swarm optimization and inclined block rate.Keywords
Cite This Article
Citations
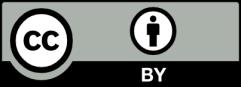