Open Access
ARTICLE
A Cyber Kill Chain Approach for Detecting Advanced Persistent Threats
1 School of Computing and Digital Technology, Birmingham City University, Birmingham, UK
2 Faculty of Computing, IBM CoE, ERAS, University Malaysia Pahang, Pahang, Malaysia
* Corresponding Author: Yussuf Ahmed. Email:
(This article belongs to the Special Issue: Machine Learning-based Secured and Privacy-preserved Smart City)
Computers, Materials & Continua 2021, 67(2), 2497-2513. https://doi.org/10.32604/cmc.2021.014223
Received 07 September 2020; Accepted 13 December 2020; Issue published 05 February 2021
Abstract
The number of cybersecurity incidents is on the rise despite significant investment in security measures. The existing conventional security approaches have demonstrated limited success against some of the more complex cyber-attacks. This is primarily due to the sophistication of the attacks and the availability of powerful tools. Interconnected devices such as the Internet of Things (IoT) are also increasing attack exposures due to the increase in vulnerabilities. Over the last few years, we have seen a trend moving towards embracing edge technologies to harness the power of IoT devices and 5G networks. Edge technology brings processing power closer to the network and brings many advantages, including reduced latency, while it can also introduce vulnerabilities that could be exploited. Smart cities are also dependent on technologies where everything is interconnected. This interconnectivity makes them highly vulnerable to cyber-attacks, especially by the Advanced Persistent Threat (APT), as these vulnerabilities are amplified by the need to integrate new technologies with legacy systems. Cybercriminals behind APT attacks have recently been targeting the IoT ecosystems, prevalent in many of these cities. In this paper, we used a publicly available dataset on Advanced Persistent Threats (APT) and developed a data-driven approach for detecting APT stages using the Cyber Kill Chain. APTs are highly sophisticated and targeted forms of attacks that can evade intrusion detection systems, resulting in one of the greatest current challenges facing security professionals. In this experiment, we used multiple machine learning classifiers, such as Naïve Bayes, Bayes Net, KNN, Random Forest and Support Vector Machine (SVM). We used Weka performance metrics to show the numeric results. The best performance result of 91.1% was obtained with the Naïve Bayes classifier. We hope our proposed solution will help security professionals to deal with APTs in a timely and effective manner.Keywords
Cite This Article
Citations
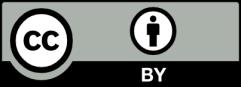
This work is licensed under a Creative Commons Attribution 4.0 International License , which permits unrestricted use, distribution, and reproduction in any medium, provided the original work is properly cited.