Open Access
ARTICLE
Exploiting Deep Learning Techniques for Colon Polyp Segmentation
1 Department of Computer Science and Engineering, University of Louisville, Louisville, KY, USA
2 Centro de Computacion Cientifica Apolo at Universidad EAFIT, Medelin, Colombia
3 eVida Research Group, University of Deusto, Bilbao, Spain
* Corresponding Author: Daniel Sierra-Sosa. Email:
(This article belongs to the Special Issue: AI, IoT, Blockchain Assisted Intelligent Solutions to Medical and Healthcare Systems)
Computers, Materials & Continua 2021, 67(2), 1629-1644. https://doi.org/10.32604/cmc.2021.013618
Received 13 August 2020; Accepted 10 November 2020; Issue published 05 February 2021
Abstract
As colon cancer is among the top causes of death, there is a growing interest in developing improved techniques for the early detection of colon polyps. Given the close relation between colon polyps and colon cancer, their detection helps avoid cancer cases. The increment in the availability of colorectal screening tests and the number of colonoscopies have increased the burden on the medical personnel. In this article, the application of deep learning techniques for the detection and segmentation of colon polyps in colonoscopies is presented. Four techniques were implemented and evaluated: Mask-RCNN, PANet, Cascade R-CNN and Hybrid Task Cascade (HTC). These were trained and tested using CVC-Colon database, ETIS-LARIB Polyp, and a proprietary dataset. Three experiments were conducted to assess the techniques performance: 1) Training and testing using each database independently, 2) Mergingd the databases and testing on each database independently using a merged test set, and 3) Training on each dataset and testing on the merged test set. In our experiments, PANet architecture has the best performance in Polyp detection, and HTC was the most accurate to segment them. This approach allows us to employ Deep Learning techniques to assist healthcare professionals in the medical diagnosis for colon cancer. It is anticipated that this approach can be part of a framework for a semi-automated polyp detection in colonoscopies.Keywords
Cite This Article
D. Sierra-Sosa, S. Patino-Barrientos, B. Garcia-Zapirain, C. Castillo-Olea and A. Elmaghraby, "Exploiting deep learning techniques for colon polyp segmentation," Computers, Materials & Continua, vol. 67, no.2, pp. 1629–1644, 2021. https://doi.org/10.32604/cmc.2021.013618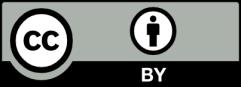