Open Access
ARTICLE
Diabetes Type 2: Poincaré Data Preprocessing for Quantum Machine Learning
1 Department of Computer Science and Engineering, University of Louisville, Louisville, KY, USA
2 Apolo Scientific Computing Center, Universidad EAFIT, Medellín, Colombia
3 eVida Research Group, University of Deusto, Bilbao, Spain
* Corresponding Author: Daniel Sierra-Sosa. Email:
(This article belongs to the Special Issue: AI, IoT, Blockchain Assisted Intelligent Solutions to Medical and Healthcare Systems)
Computers, Materials & Continua 2021, 67(2), 1849-1861. https://doi.org/10.32604/cmc.2021.013196
Received 17 July 2020; Accepted 07 December 2020; Issue published 05 February 2021
Abstract
Quantum Machine Learning (QML) techniques have been recently attracting massive interest. However reported applications usually employ synthetic or well-known datasets. One of these techniques based on using a hybrid approach combining quantum and classic devices is the Variational Quantum Classifier (VQC), which development seems promising. Albeit being largely studied, VQC implementations for “real-world” datasets are still challenging on Noisy Intermediate Scale Quantum devices (NISQ). In this paper we propose a preprocessing pipeline based on Stokes parameters for data mapping. This pipeline enhances the prediction rates when applying VQC techniques, improving the feasibility of solving classification problems using NISQ devices. By including feature selection techniques and geometrical transformations, enhanced quantum state preparation is achieved. Also, a representation based on the Stokes parameters in the Poincaré Sphere is possible for visualizing the data. Our results show that by using the proposed techniques we improve the classification score for the incidence of acute comorbid diseases in Type 2 Diabetes Mellitus patients. We used the implemented version of VQC available on IBM’s framework Qiskit, and obtained with two and three qubits an accuracy of 70% and 72% respectively.Keywords
Cite This Article
Citations
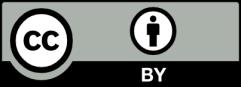
This work is licensed under a Creative Commons Attribution 4.0 International License , which permits unrestricted use, distribution, and reproduction in any medium, provided the original work is properly cited.