Open Access
ARTICLE
An Online Chronic Disease Prediction System Based on Incremental Deep Neural Network
1 School of Design, Jiangnan University, Wuxi, 214122, China
2 School of Computer and Communication Engineering, Changsha University of Science and Technology, Changsha, 410114, China
3 Jiangsu Engineering Center of Network Monitoring, Nanjing University of Information Science and Technology, Nanjing, 210044, China
4 School of Electrical and Data Engineering, University of Technology Sydney, Sydney, 2000, Australia
* Corresponding Author: Bin Yang. Email:
Computers, Materials & Continua 2021, 67(1), 951-964. https://doi.org/10.32604/cmc.2021.014839
Received 21 October 2020; Accepted 12 November 2020; Issue published 12 January 2021
Abstract
Many chronic disease prediction methods have been proposed to predict or evaluate diabetes through artificial neural network. However, due to the complexity of the human body, there are still many challenges to face in that process. One of them is how to make the neural network prediction model continuously adapt and learn disease data of different patients, online. This paper presents a novel chronic disease prediction system based on an incremental deep neural network. The propensity of users suffering from chronic diseases can continuously be evaluated in an incremental manner. With time, the system can predict diabetes more and more accurately by processing the feedback information. Many diabetes prediction studies are based on a common dataset, the Pima Indians diabetes dataset, which has only eight input attributes. In order to determine the correlation between the pathological characteristics of diabetic patients and their daily living resources, we have established an in-depth cooperation with a hospital. A Chinese diabetes dataset with 575 diabetics was created. Users’ data collected by different sensors were used to train the network model. We evaluated our system using a real-world diabetes dataset to confirm its effectiveness. The experimental results show that the proposed system can not only continuously monitor the users, but also give early warning of physiological data that may indicate future diabetic ailments.Keywords
Cite This Article
Citations
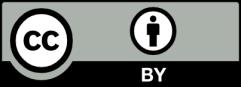
This work is licensed under a Creative Commons Attribution 4.0 International License , which permits unrestricted use, distribution, and reproduction in any medium, provided the original work is properly cited.