Open Access
ARTICLE
SwCS: Section-Wise Content Similarity Approach to Exploit Scientific Big Data
1 Department of Computer Science, Capital University of Science and Technology, Islamabad, Pakistan
2 Department of Computer Science, NAMAL Institute, Mianwali, 42250, Pakistan
3 Raptor Interactive (Pty) Ltd., Eco Boulevard, Witch Hazel Ave, Centurion, 0157, Africa
4 Institute of Computing, Kohat University of Science and Technology, Pakistan
5 Department of CS&IT, University of Azad Jammu and Kashmir, Muzaffarabad, 13100, Pakistan
6 Department of Artificial Intelligence, Ajou University, Korea
* Corresponding Author: Tae-Sun Chung. Email:
(This article belongs to the Special Issue: Artificial Intelligence and Big Data in Entrepreneurship)
Computers, Materials & Continua 2021, 67(1), 877-894. https://doi.org/10.32604/cmc.2021.014156
Received 02 September 2020; Accepted 28 October 2020; Issue published 12 January 2021
Abstract
The growing collection of scientific data in various web repositories is referred to as Scientific Big Data, as it fulfills the four “V’s” of Big Data–-volume, variety, velocity, and veracity. This phenomenon has created new opportunities for startups; for instance, the extraction of pertinent research papers from enormous knowledge repositories using certain innovative methods has become an important task for researchers and entrepreneurs. Traditionally, the content of the papers are compared to list the relevant papers from a repository. The conventional method results in a long list of papers that is often impossible to interpret productively. Therefore, the need for a novel approach that intelligently utilizes the available data is imminent. Moreover, the primary element of the scientific knowledge base is a research article, which consists of various logical sections such as the Abstract, Introduction, Related Work, Methodology, Results, and Conclusion. Thus, this study utilizes these logical sections of research articles, because they hold significant potential in finding relevant papers. In this study, comprehensive experiments were performed to determine the role of the logical sections-based terms indexing method in improving the quality of results (i.e., retrieving relevant papers). Therefore, we proposed, implemented, and evaluated the logical sections-based content comparisons method to address the research objective with a standard method of indexing terms. The section-based approach outperformed the standard content-based approach in identifying relevant documents from all classified topics of computer science. Overall, the proposed approach extracted 14% more relevant results from the entire dataset. As the experimental results suggested that employing a finer content similarity technique improved the quality of results, the proposed approach has led the foundation of knowledge-based startups.Keywords
Cite This Article
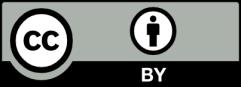