Open Access
ARTICLE
A Fast and Effective Multiple Kernel Clustering Method on Incomplete Data
1 Changsha Hunan Provincial Key Laboratory of Intelligent Processing of Big Data on Transportation, School of Computer and Communication Engineering, Changsha University of Science and Technology, Changsha, 410114, China
2 Hunan Key Laboratory of Smart Roadway and Cooperative Vehicle-Infrastructure Systems, Changsha University of Science and Technology, Changsha, 410114, China
3 Faculty of Engineering and Information Technology, Global Big Data Technologies Centre, University of Technology Sydney, Ultimo, NSW, 2007, Australia
4 Department of Computer Engineering, Chonnam National University, Gwangju, 61186, Korea
5 Computer Science Department, Community College, King Saud University, Riyadh, 11437, Saudi Arabia
6 Department of Mathematics and Computer Science, Faculty of Science, Menoufia University, Shebin-El-kom, 32511, Egypt
* Corresponding Author: Gwang-Jun Kim. Email:
Computers, Materials & Continua 2021, 67(1), 267-284. https://doi.org/10.32604/cmc.2021.013488
Received 08 August 2020; Accepted 12 September 2020; Issue published 12 January 2021
Abstract
Multiple kernel clustering is an unsupervised data analysis method that has been used in various scenarios where data is easy to be collected but hard to be labeled. However, multiple kernel clustering for incomplete data is a critical yet challenging task. Although the existing absent multiple kernel clustering methods have achieved remarkable performance on this task, they may fail when data has a high value-missing rate, and they may easily fall into a local optimum. To address these problems, in this paper, we propose an absent multiple kernel clustering (AMKC) method on incomplete data. The AMKC method first clusters the initialized incomplete data. Then, it constructs a new multiple-kernel-based data space, referred to as K-space, from multiple sources to learn kernel combination coefficients. Finally, it seamlessly integrates an incomplete-kernel-imputation objective, a multiple-kernel-learning objective, and a kernel-clustering objective in order to achieve absent multiple kernel clustering. The three stages in this process are carried out simultaneously until the convergence condition is met. Experiments on six datasets with various characteristics demonstrate that the kernel imputation and clustering performance of the proposed method is significantly better than state-of-the-art competitors. Meanwhile, the proposed method gains fast convergence speed.Keywords
Cite This Article
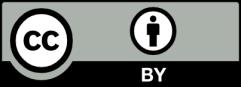
This work is licensed under a Creative Commons Attribution 4.0 International License , which permits unrestricted use, distribution, and reproduction in any medium, provided the original work is properly cited.