Open Access
ARTICLE
Intelligent Fusion of Infrared and Visible Image Data Based on Convolutional Sparse Representation and Improved Pulse-Coupled Neural Network
1 School of Artificial Intelligence, Nanjing University of Information Science & Technology, Nanjing, 210044, China
2 School of Computer and Software, Nanjing University of Information Science & Technology, Nanjing, 210044, China
3 Western University, London, N6A 3K7, Canada
* Corresponding Author: Ling Tan. Email:
Computers, Materials & Continua 2021, 67(1), 613-624. https://doi.org/10.32604/cmc.2021.013457
Received 07 August 2020; Accepted 12 September 2020; Issue published 12 January 2021
Abstract
Multi-source information can be obtained through the fusion of infrared images and visible light images, which have the characteristics of complementary information. However, the existing acquisition methods of fusion images have disadvantages such as blurred edges, low contrast, and loss of details. Based on convolution sparse representation and improved pulse-coupled neural network this paper proposes an image fusion algorithm that decompose the source images into high-frequency and low-frequency subbands by non-subsampled Shearlet Transform (NSST). Furthermore, the low-frequency subbands were fused by convolutional sparse representation (CSR), and the high-frequency subbands were fused by an improved pulse coupled neural network (IPCNN) algorithm, which can effectively solve the problem of difficulty in setting parameters of the traditional PCNN algorithm, improving the performance of sparse representation with details injection. The result reveals that the proposed method in this paper has more advantages than the existing mainstream fusion algorithms in terms of visual effects and objective indicators.Keywords
Cite This Article
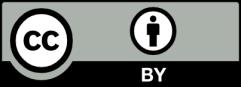
This work is licensed under a Creative Commons Attribution 4.0 International License , which permits unrestricted use, distribution, and reproduction in any medium, provided the original work is properly cited.