Open Access
ARTICLE
Geospatial Analytics for COVID-19 Active Case Detection
1 Multimedia University, Cyberjaya, 63100, Malaysia
2 AIME Healthcare Sdn Bhd, Kuala Lumpur, 59200, Malaysia
* Corresponding Author: Choo-Yee Ting. Email:
(This article belongs to the Special Issue: Machine Learning and Computational Methods for COVID-19 Disease Detection and Prediction)
Computers, Materials & Continua 2021, 67(1), 835-848. https://doi.org/10.32604/cmc.2021.013327
Received 02 August 2020; Accepted 12 November 2020; Issue published 12 January 2021
Abstract
Ever since the COVID-19 pandemic started in Wuhan, China, much research work has been focusing on the clinical aspect of SARS-CoV-2. Researchers have been leveraging on various Artificial Intelligence techniques as an alternative to medical approach in understanding the virus. Limited studies have, however, reported on COVID-19 transmission pattern analysis, and using geography features for prediction of potential outbreak sites. Predicting the next most probable outbreak site is crucial, particularly for optimizing the planning of medical personnel and supply resources. To tackle the challenge, this work proposed distance-based similarity measures to predict the next most probable outbreak site together with its magnitude, when would the outbreak likely to happen and the duration of the outbreak. The work began with preprocessing of 1365 patient records from six districts in the most populated state named Selangor in Malaysia. The dataset was then aggregated with population density information and human elicited geography features that might promote the transmission of COVID-19. Empirical findings indicated that the proposed unified decision-making approach outperformed individual distance metric in predicting the total cases, next outbreak location, and the time interval between start dates of two similar sites. Such findings provided valuable insights for policymakers to perform Active Case Detection.Keywords
Cite This Article
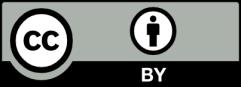
This work is licensed under a Creative Commons Attribution 4.0 International License , which permits unrestricted use, distribution, and reproduction in any medium, provided the original work is properly cited.